Image
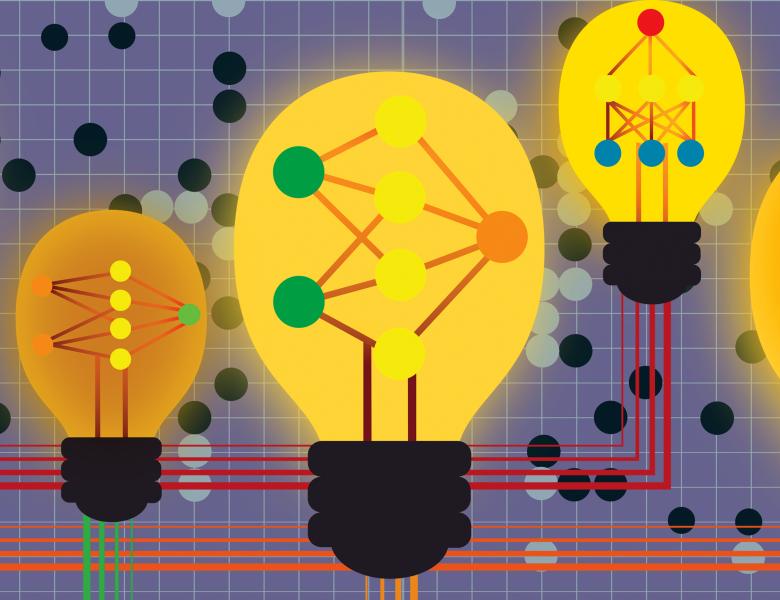
In this talk I will show that Learning Automata (LA), and more precisely Reward in Action update schemes are interesting building blocks for Multi-agent RL, both in bandit settings as well as stateful RL. Based on the theorem of Narendra and Wheeler we have convergence guarantees in n-person non-zero sum games. However, LA have also shown to be robust in more relaxed settings, such as queueing systems, where updates happen asynchronously and the feedback sent to the agents is delayed.