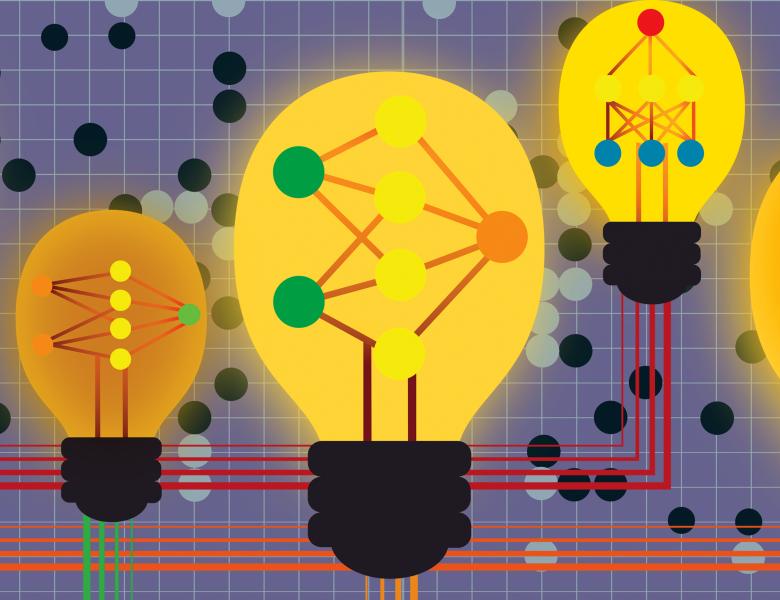
Abstract
The learning in games literature interprets equilibrium strategy profiles as the long-run average behavior of agents who are selected at random to play the game. In normal-form games we expect that as the agents accumulate evidence about play of the game they will develop accurate beliefs, so that the stationary points of the process correspond to the Nash equilibria. There is no reason to expect learning by myopic agents to lead to Nash equilibrium in general games, as agents may not experiment enough to learn the consequences of deviating from the equilibrium path. The focus here is on settings where the agents are patient, so they do have an incentive to experiment, and stationary points must ne Nash equilibria.However, eExtensive-form games typically have many Nash equilibria, and not all of them seem equally plausible. This talk discusses the restrictions that learning models impose on Nash equilibria and how these differ from the restrictions of classical equilibrium refinements. This talk discusses the restrictions that learning models impose on Nash equilibria and how these differ from the restrictions of classical equilibrium refinements.