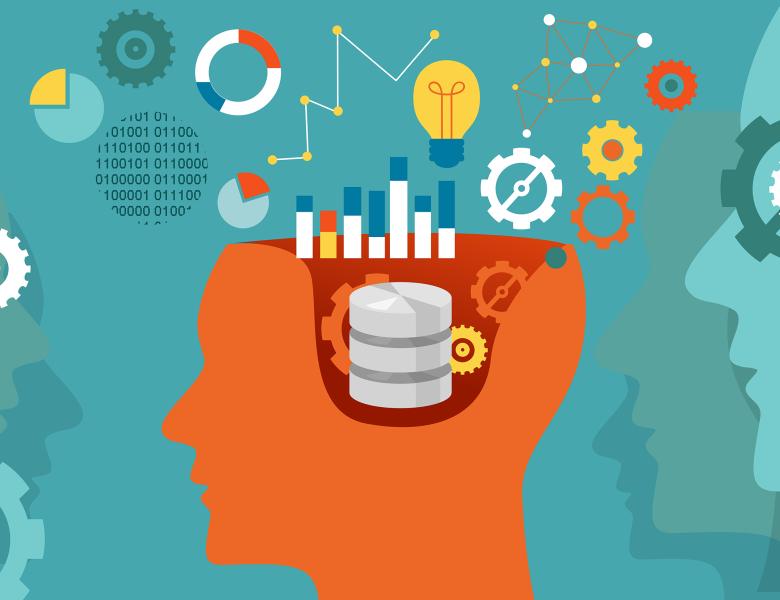
Abstract
Recent work on supervised learning [GKRSW22] defined the notion of omnipredictors, i.e., predictor functions p over features that are simultaneously competitive for minimizing a family of loss functions L against a comparator class C. Omniprediction requires approximating the Bayes-optimal predictor beyond the loss minimization paradigm, and has generated significant interest in the learning theory community. However, even for basic settings such as agnostically learning single-index models (SIMs), existing omnipredictor constructions require impractically-large sample complexities and runtimes, and output complex, highly-improper hypotheses.
Our main contribution is a new, simple construction of omnipredictors for SIMs. We give a learner outputting an omnipredictor that is eps-competitive on any matching loss induced by a monotone, Lipschitz link function, when the comparator class is bounded linear predictors. Our algorithm requires roughly eps^-4 samples and runs in nearly-linear time, and its sample complexity improves to roughly eps^-2 if link functions are bi-Lipschitz. This significantly improves upon the only prior known construction, due to [HKRR18, GHKRW23], which used at least eps^-10 samples.
We achieve our construction via a new, sharp analysis of the classical Isotron algorithm [KS09, KKKS11] in the challenging agnostic learning setting, of potential independent interest. Previously, Isotron was known to properly learn SIMs in the realizable setting, as well as constant-factor competitive hypotheses under the squared loss [ZWDD24]. As they are based on Isotron, our omnipredictors are multi-index models with eps^-2 prediction heads, bringing us closer to the tantalizing goal of proper omniprediction for general loss families and comparators.
Joint work with Lunjia Hu and Chutong Yang.