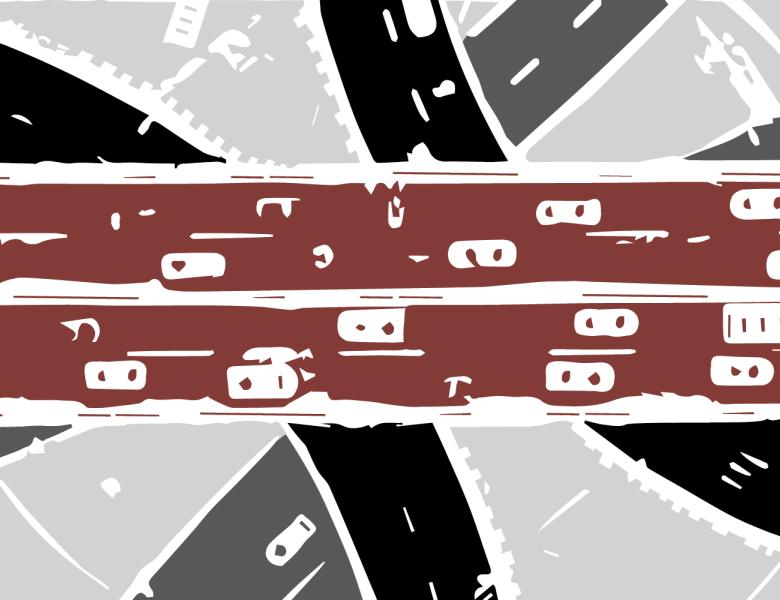
Abstract
I describe efforts to apply statistical machine learning to large-scale astronomy datasets both in batch and streaming mode. For the past decade, feature-engineering-based approaches applied to the discovery of supernovae and the characterization of tens of thousands of variable stars led the way to novel astronomical inference. Here I will show that new auto-encoder recurrent neural network architectures, without hand-crafted features, rival those traditional methods. Autonomous discovery and inference are part of a larger worldwide onus to federate precious (and heterogeneous) follow-up resources to maximize our collective scientific returns.