Image
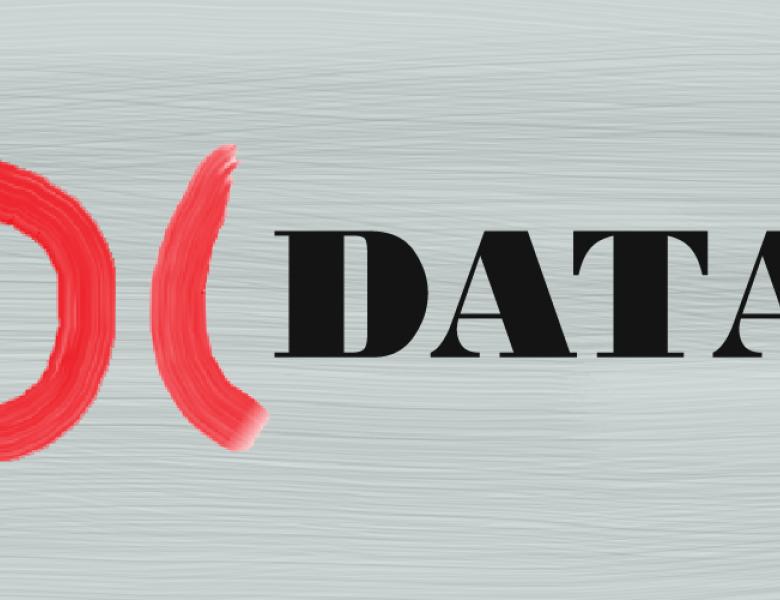
Principal components analysis is a popular dimension reduction method with wide applications. In high dimensional data analysis, sparse PCA offers simultaneous dimension reduction and variable selection. This talk will report some recent development in sparse PCA with a special focus on subspace estimation. The results include minimax rates of estimation, a new convex relaxation, and some statistical properties of this new method such as matrix norm convergence and variable selection consistency. Some possible future research topics related to differential privacy will also be mentioned.