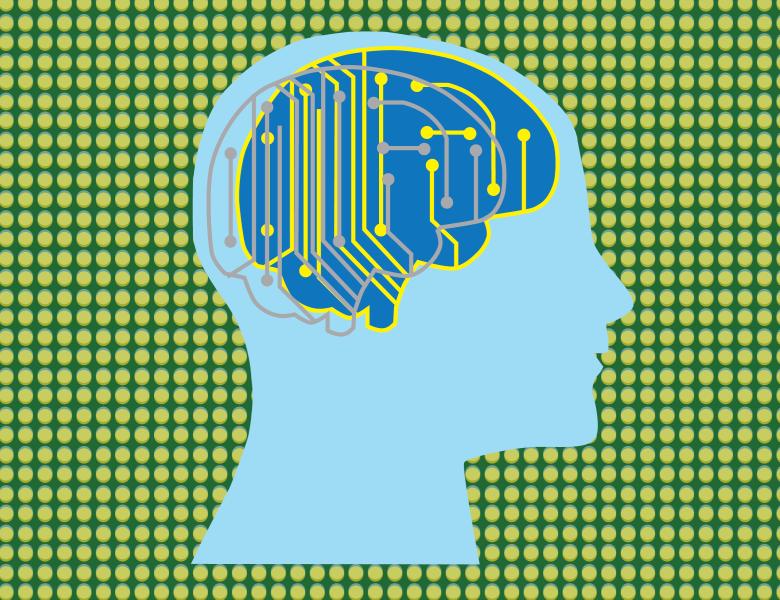
Abstract
Neuroscience is experiencing a data revolution in which simultaneous recording of many hundreds or thousands of neurons is revealing structure in population activity that is not apparent from single-neuron responses. This structure is typically extracted from trial-averaged data. Single-trial analyses are challenging due to incomplete sampling of the neural population, trial-to-trial variability, and fluctuations in action potential timing. Here we introduce Latent Factor Analysis via Dynamical Systems (LFADS), a deep learning method to infer latent dynamics from single-trial neural spiking data. LFADS uses a nonlinear dynamical system (a recurrent neural network) to infer the dynamics underlying observed population activity and to extract ‘de-noised’ single-trial firing rates from neural spiking data. We apply LFADS to a variety of monkey and human motor cortical datasets, demonstrating its ability to predict observed behavioral variables with unprecedented accuracy, extract precise estimates of neural dynamics on single trials, infer perturbations to those dynamics that correlate with behavioral choices, and combine data from non-overlapping recording sessions (spanning months) to improve inference of underlying dynamics. In summary, LFADS leverages all observations of a neural population's activity to accurately model its dynamics on single trials, opening the door to a detailed understanding of the role of dynamics in performing computation and ultimately driving behavior.