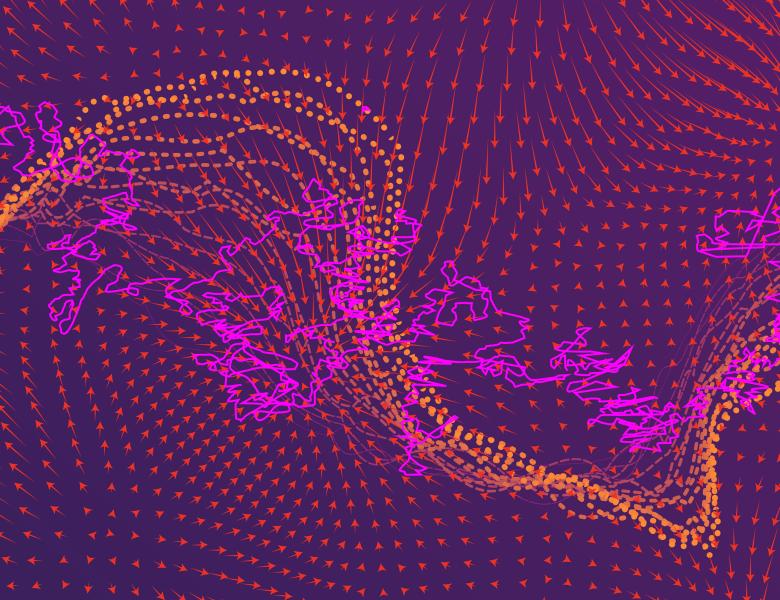
Abstract
The popular diffusion scaling heuristic predicts that the mixing time of the Metropolis-Adjusted Langevin Algorithm (MALA) should scale as d^{1/3}, where d is the dimension. However, the diffusion scaling limit requires stringent assumptions and is asymptotic in nature. In the first part of my talk, I will discuss our work on improving the state-of-the-art non-asymptotic mixing time bound for MALA on the class of log-smooth and strongly log-concave distributions from O(d) to O(d^{1/2}), under the additional assumption of a warm start. Our proof introduces a new technique based on a projection characterization of the Metropolis adjustment which reduces the study of MALA to the discretization analysis of the Langevin SDE. In the second part of my talk, I will discuss the elusive problem of obtaining lower bounds for sampling, including our recent work which establishes the first tight query complexity bound for sampling in one dimension.