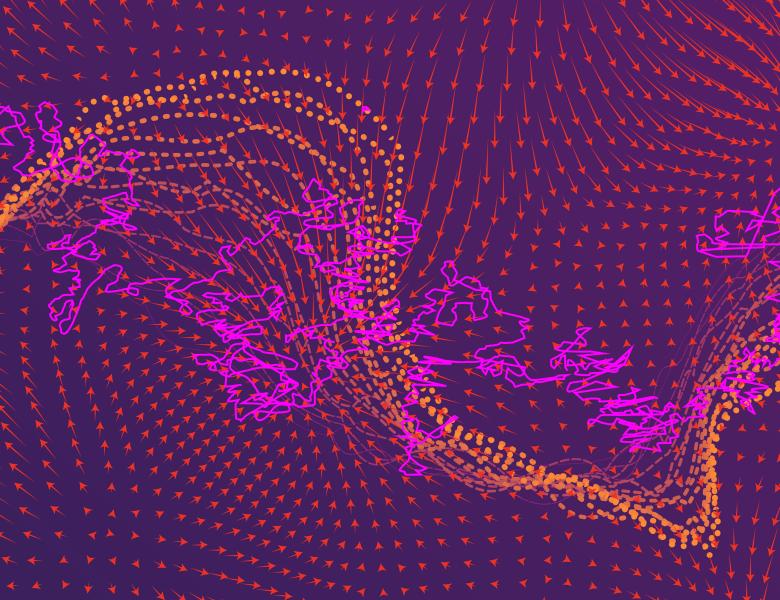
Abstract
Clustering data generated by a mixture of pdf's is an important problem which has received a lot of attention. In most algorithms, k, the number of clusters is assumed to be given. In addition to being crucial in defining the objective functions like k-means, the value of k dictates which data points belong to a single cluster. We develop the first provable polynomial time algorithm for finding k from data under general deterministic conditions. Our starting point is a geometric condition we call No Tight Sub-Cluster (NTSC) based on the spectral norm of a normalized data matrix, which defines what constitutes a cluster. We show that if all 1-dimensional marginals of a pdf satisfy a pointwise upper bound (pub), then data generated by the pdf satisfies NTSC. Indeed, all log-concave pdf's satisfy pub and so are special cases of our results. Our first algorithmic contribution is a polynomial time approximate check of NTSC. The harder part is to identify the clusters which involves an SDP relaxation followed by a new rounding procedure based on Cheeger's inequality.
Joint with C. Bhattacharyya, Indian Institute of Science, A. Kumar of Indian Institute of Technology, Delhi.