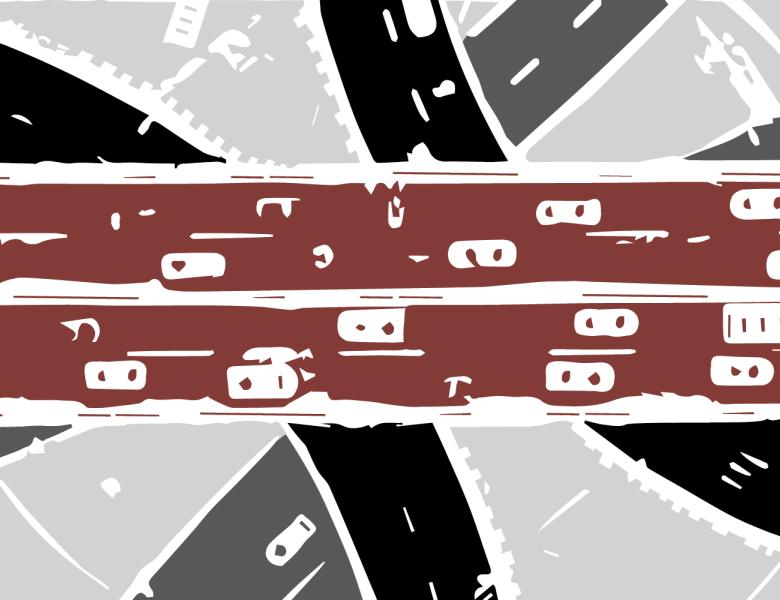
Abstract
The US has three cities in the top five congested cities in the world - which accounts for a collective economic cost of $62bn for just these three cities. Designing transportation solutions for real-world urban scale systems has previously been accomplished with very limited analytics because of the computational scale. New large scale computational capabilities (e.g. cloud computing and supercomputing), data analytics (e.g machine learning and intelligent data compression) and modeling (e.g. dynamic traffic assignment and agent-based modeling) that scale in both time and space are now possible. By combining massive amounts of data from real-world sensors and very large road network models, both closed form analytics and emergent behaviors from large scale agent models can be used to build our understanding of urban scale problems. This discussion will focus on (1) the real-world issues of handling geospatial data at scale, the data veracity issues and intelligent data compression opportunities and (2) the use of large-scale computing to address urban scale dynamic models that can be used to examine emergent behavior under a variety of conditions.