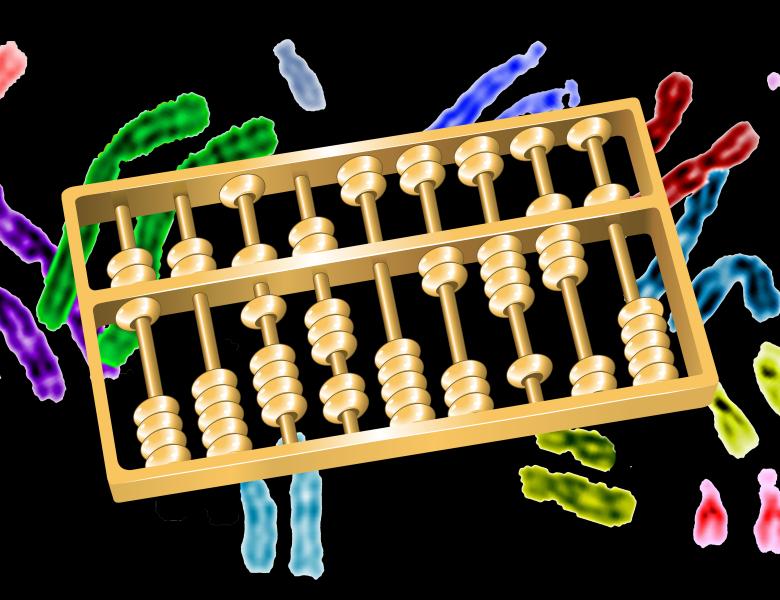
Abstract
The Encyclopedia of DNA Elements (ENCODE) Consortium has generated tens of thousands of high-throughput genomic datasets with the goal of cataloging all of the functional elements of the human genome. Now, our goal is to integrate these complex data types to annotate regulatory elements such as enhancers and create an encyclopedia of elements for the human and mouse research communities.
We began by analyzing enhancer prediction methods. We tested many different models incorporating data such as DNase-seq, histone mark ChIP-seq, and DNA methylation. We evaluated our methods using experimentally validated enhancer regions from the VISTA enhancer database on four embryonic mouse tissues: limb, hindbrain, midbrain, and neural tube. Overall, the best performing method was centering predictions on DNase peaks and ranking these peaks by the average rank of DNAse and H3K27ac signal. We then applied this method to all mouse and human cell types in ENCODE.
After identifying candidate enhancers, we next sought to identifying the target genes of these regions. In order to evaluate different methods, we created training/validation/test datasets from promoter capture Hi-C datasets in GM12878. We began by analyzing correlation based methods where enhancer-gene links are predicted by high correlation of DNase or H3K27ac signal across multiple cell types. While these methods have previously been used in the literature, we found that they performed poorly (AUROC=0.6 , AUPR=0.06). We then decided to use a Random Forest based approach which would incorporate additional data such as distance between the gene and enhancer, average DNase and H3K27ac signals as well as correlation. Though model had a substantial increase in performance (AUROC=0.78, AUPR=0.16) there is still a great deal of improvement that can be made. We hope to add additional features to our model as well as find the best performing model with limited features that can be applied across many different ENCODE cell types.