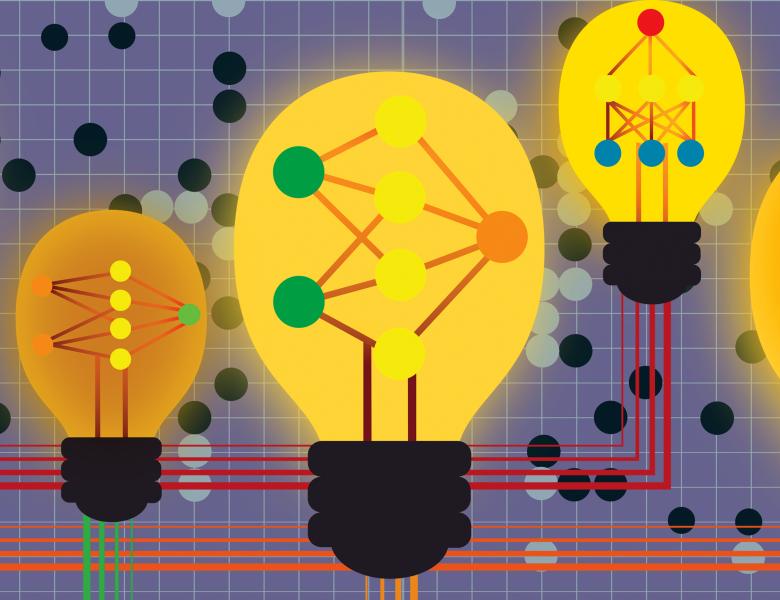
Abstract
Regret minimizing agents in self-play have been used to learn approximate minimax-optimal strategies with much success, scaling to large hold’em poker games and to super-human level performance in very large multiplayer games. This prescriptive approach has guided the development of algorithms for two-player zero-sum games, and similarly for fully-cooperative games. What about the fully general case– what could a prescriptive agenda look like there? Is there an agent-centric criterion that can be optimized without relying on outside authorities or third parties? In this talk, I will quickly survey the recent approaches to game-theoretic multiagent reinforcement learning in general games, and then focus on ideas that could attempt to answer these open questions in multiagent reinforcement learning.