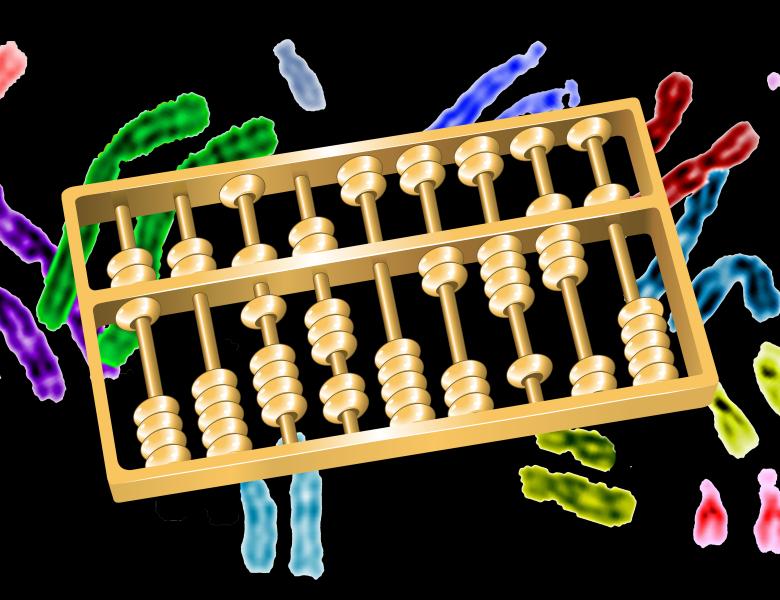
Abstract
Non-coding variants implicated in genome-wide association studies (GWAS) are enriched in enhancer elements active in disease-relevant cellular contexts. Identifying context-specific target genes and downstream pathways affected by enhancers harboring regulatory variants remains a challenge. We develop novel learning algorithms that leverage the modular dynamics of gene expression and enhancer associated chromatin marks across a vast collection of diverse human cell types and tissues from the ENCODE and Roadmap Epigenomics Projects to infer highly-connected, context-specific enhancer-gene networks. Chromatin conformation maps and expression QTLs validate the superior accuracy and tissue-specificity of our predicted networks compared to existing approaches. We find that a significant proportion of enhancers do not associate with their nearest genes indicating pervasive distal regulation potentially mediated by long-range chromatin contacts. Linked enhancers significantly improve tissue-specific regression models of gene expression. Distal co-association of regulatory sequence motifs suggests synergistic regulation of genes by multiple enhancers with a key role for protein-protein interactions between lineage-specific transcription factors in mediating enhancer-promoter interactions. Networks of cooperating enhancers with shared motif composition and target genes are depleted of disease-associated variants, suggesting regulatory buffering mechanisms. We demonstrate the utility of our context-specific enhancer-gene links to predict putative target genes, biological processes and pathways of non-coding variants associated with diverse traits and diseases