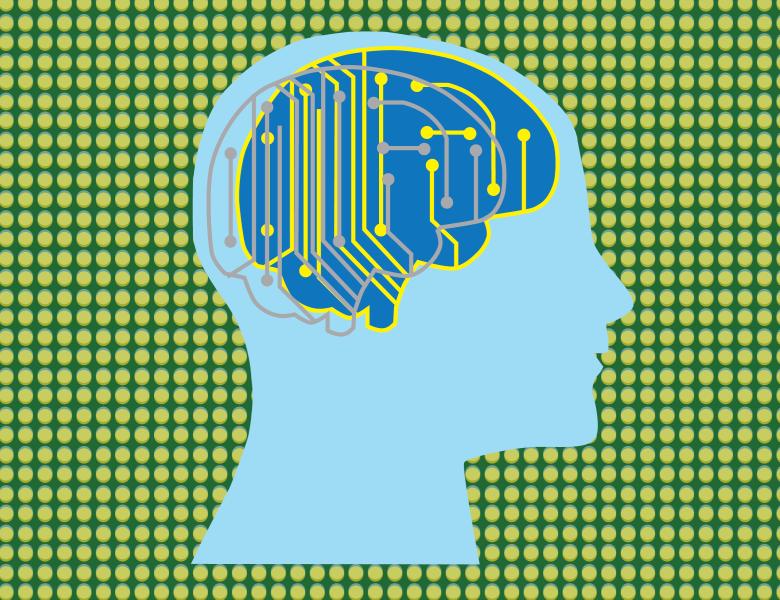
Abstract
Our brain’s functions and dysfunctions arise from neural activity at multiple spatiotemporal scales, from small-scale spikes of individual neurons to large-scale network activity measured through local field potentials (LFP) and electrocorticogram. Thus, developing new algorithms that can simultaneously model and decode multiple scales of activity is important for understanding neural mechanisms. Moreover, designing model-based algorithms for closed-loop control of neural activity with stimulation input is critical for establishing functional connectivity in neural circuits and devising precisely-tailored therapies for neurological disorders. We discuss some of our recent work on developing algorithms for modelling, decoding, and control of multiscale neural activity. We first present a multiscale dynamical modelling framework that identifies a unified low-dimensional latent state from hybrid spike-field activity. We show that the framework can combine information from multiple scales of activity recorded from monkeys, and model the different time-scales and statistical profiles across these scales. We then demonstrate that this framework allows us, for the first time, to decode mood variations over time from distributed multisite human brain activity. Further, we identify brain sites that are most predictive of human mood states. Finally, we present a new control-theoretic system-identification approach to characterize brain network dynamics (output) in response to electrical and optogenetic stimulation (input) within our dynamical model. To collect optimal input-output data for model estimation, we design a novel input waveform—a pulse train modulated by binary noise (BN) parameters such as pulse frequency—that we show is optimal for system-identification and conforms to clinical safety requirements. We apply the BN electrical and optogenetic stimulation waveforms in human and monkey experiments, respectively. We show that our estimated models can accurately predict both the dynamic and the steady-state neural response to stimulation input. These results show the feasibility of inferring complex brain states from distributed multiscale activity and identifying predictive dynamic models of neural response to stimulation. These algorithms could facilitate future closed-loop therapies for neurological disorders and help probe neural circuits.