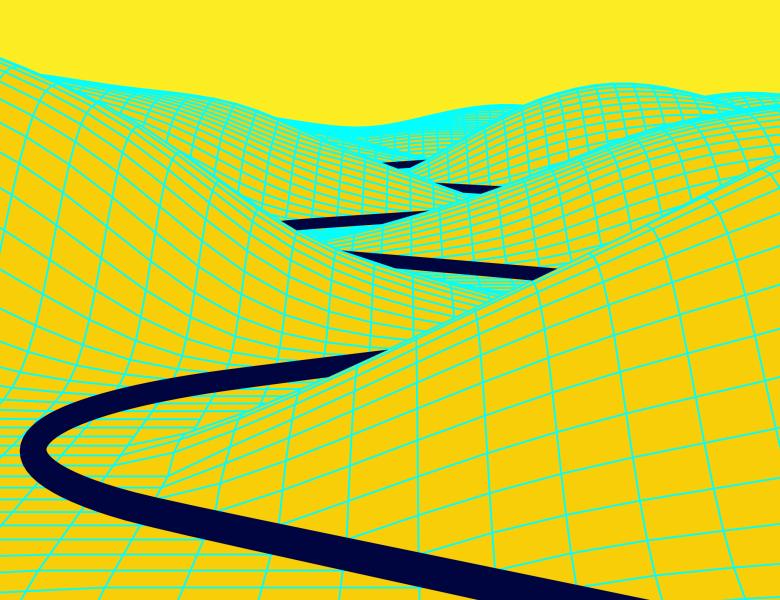
Abstract
Abstract: In this talk, I shall present two research vignettes on the generalization of interpolating models.
Prior work has presented strong empirical evidence demonstrating that importance weights can have little to no effect on interpolating neural networks. We show that importance weighting fails not because of the interpolation, but instead, as a result of using exponentially-tailed losses like the cross-entropy loss. As a remedy, we show that polynomially-tailed losses restore the effects of importance reweighting in correcting distribution shift in interpolating models trained by gradient descent. Surprisingly, our theory reveals that using biased importance weights can improve performance in interpolating models.
Second, I shall present lower bounds on the excess risk of sparse interpolating procedures for linear regression. Our result shows that the excess risk of the minimum L1-norm interpolant can converge at an exponentially slower rate than the minimum L2-norm interpolant, even when the ground truth is sparse. Our analysis exposes the benefit of an effect analogous to the "wisdom of the crowd", except here the harm arising from fitting the noise is ameliorated by spreading it among many directions.
Based on joint work with Tatsunori Hashimoto, Saminul Haque, Philip Long, and Alexander Wang.