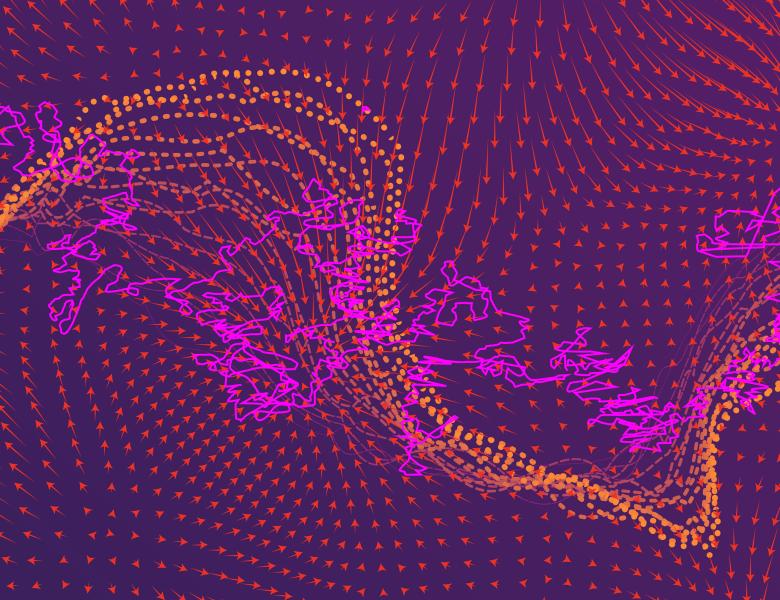
Abstract
Constraint-based reconstruction and analysis provides a molecular mechanistic framework for integrative analysis of experimental molecular systems biology data and quantitative prediction of physicochemically and biochemically feasible phenotypic states. In constraint-based modelling, physical and biochemical constraints define a set of feasible network states. When this set is polyhedral and convex, uniform sampling of is currently used to provide an unbiased characterization of the capabilities of a given biochemical network. However, reliable uniform sampling of genome-scale biochemical network models is challenging due to their high dimensionality and inherent anisotropy. Several sampling algorithms have been applied to biochemical network models and prototypical applications will be introduced. Non-uniform sampling of biochemical network models is increasingly of interest, e.g., to represent constraints arising from noisy experimental data. Emerging constraint-based modelling approaches include additional constraints resulting in either feasible sets defined by an intersection of polyhedral convex and convex cone constraints, or feasible sets that are polyhedral star convex. It is envisaged that these problems will stimulate development of novel sampling algorithms and software with widespread application in the modelling of biochemical networks.