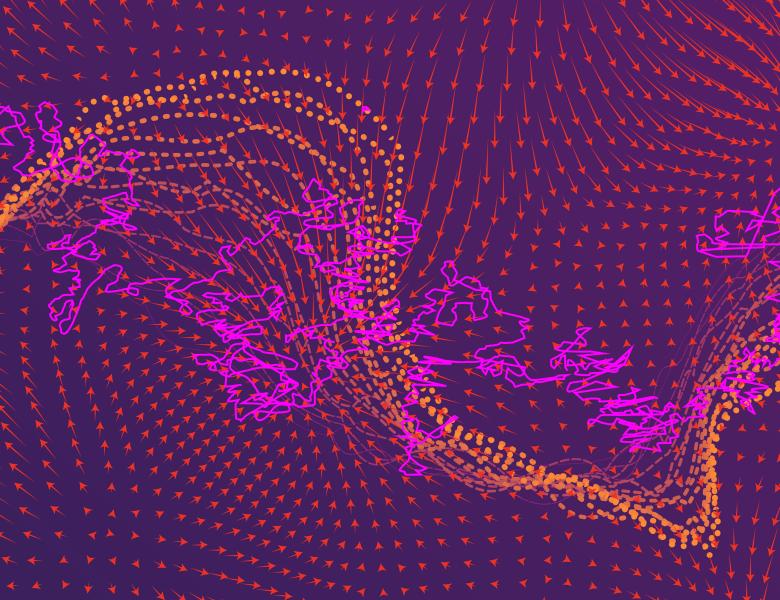
Abstract
We propose a novel method for sampling and optimization tasks based on a stochastic interacting particle system. We explain how this method can be used for the following two goals: (i) generating approximate samples from a given target distribution, and (ii) optimizing a given objective function. This approach is derivative-free and affine invariant, and is therefore well-suited for solving complex inverse problems, allowing (i) to sample from the Bayesian posterior and (ii) to find the maximum a posteriori estimator. We investigate the properties of this family of methods in terms of various parameter choices, both analytically and by means of numerical simulations. This is joint work with José A. Carrillo (University of Oxford), Andrew M. Stuart (California Institute of Technology) and Urbain Vaes (MATHERIALS team, Inria Paris).