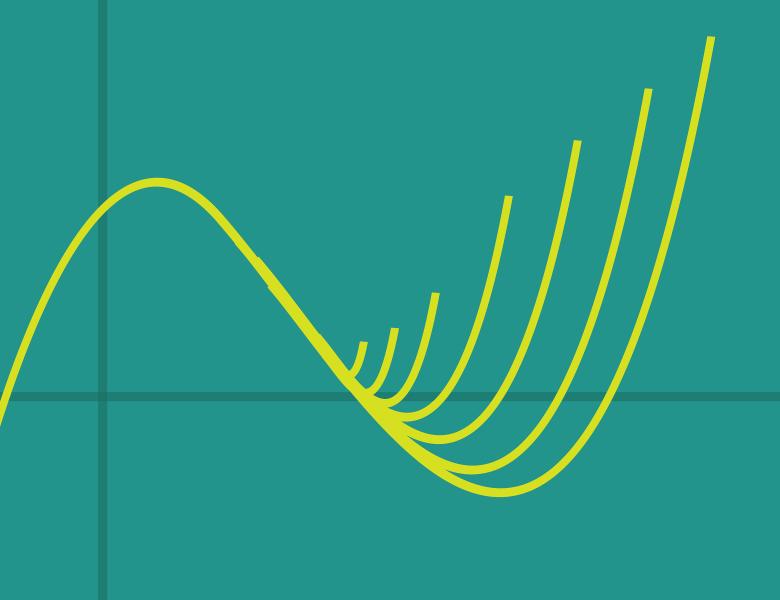
Abstract
Partition functions are a class of combinatorially derived polynomials that have many applications in statistical physics, combinatorics and machine learning. For almost all interesting partition functions exact evaluation is computationally intractable, so interest is focused on efficient approximation. The computational complexity of such approximation is often intimately related to the existence of phase transitions in the underlying physical system described by the partition function, which in turn are related to its analyticity properties. In these talks I will briefly discuss three widely applicable algorithmic techniques—Markov chain Monte Carlo, correlation decay, and polynomial interpolation—and how they relate to phase transitions, using the classical Ising model as a running example.