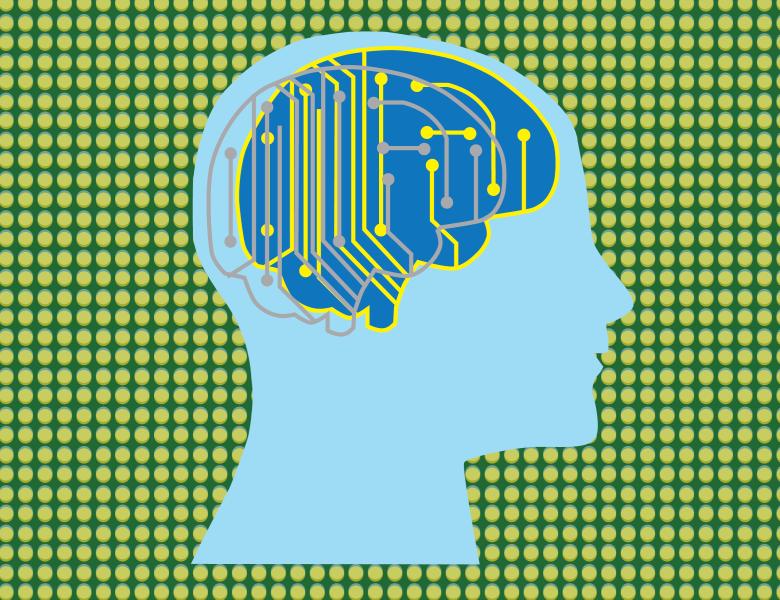
Abstract
With increasingly rich physiological and anatomical data sets, new opportunities are emerging for exploring cortical networks. For some time, multi-site recordings have been analyzed with dimensionality-reduction techniques to explore the structure of the neural code or the dynamics of neuronal ensembles. Examples include the relationship between pair-wise neuronal correlations and sensory coding; the analysis of high-order dynamical trajectories in ensemble activity; or manifold embeddings of sensory codes.
As the field of microscale connectomics matures, larger and more complete graphs of neural connectivity are becoming available. The simplest analyses of these networks have addressed the relationship between physiology and connectivity (functional connectomics), or strictly anatomical questions such as community detection. Alternatively, networks might be explored to uncover richer structures. Specifically, we have found that a manifold-embedding analysis of network connections yields surprisingly rich information about sensory representations and their transformation, even in the absence of physiological information. This approach works for both data-driven models of visual cortical networks and for the early stages of deep-learning networks, such as Alexnet.
More generally, I’ll explore the effectiveness of physiological vs. anatomical measurements for probing the structure of cortical networks; or how a combination of physiology and anatomy might be exploited.