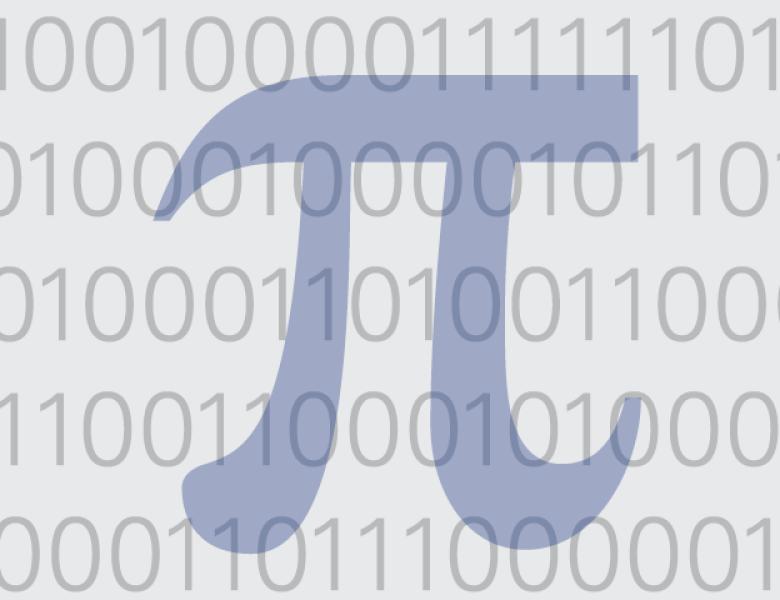
Abstract
There are a chain of connections between the boosting technique in machine learning, the hard-core distribution lemmas in complexity theory, the dense model theorems from additive combinatorics, regularity lemmas from graph theory, and back to GAN type algorithms in machine learning. Reverse engineering these connections, we consider versions of the energy increment arguments used to prove regularity lemmas as a boosting algorithm, and give a tight analysis of this algorithm using entropy. We show that it is asymptotically optimal within the class of oblivious boosting algorithms that can be used to prove the hardcore distribution lemma. We then apply the connections forward in the chain, and re-derive versions of generic regularity lemmas with (possibly) improved quantitative parameters. We show that the dependence goes from a tower to a doubly exponential function when the class of basic hypotheses have constant VC dimension. We also use the same argument to give a "decision tree'' regularity lemma with single exponential dependence on the parameters. We hope this might be usable to quantitatively improve some known consequences of the Szemeredi regularity lemma.