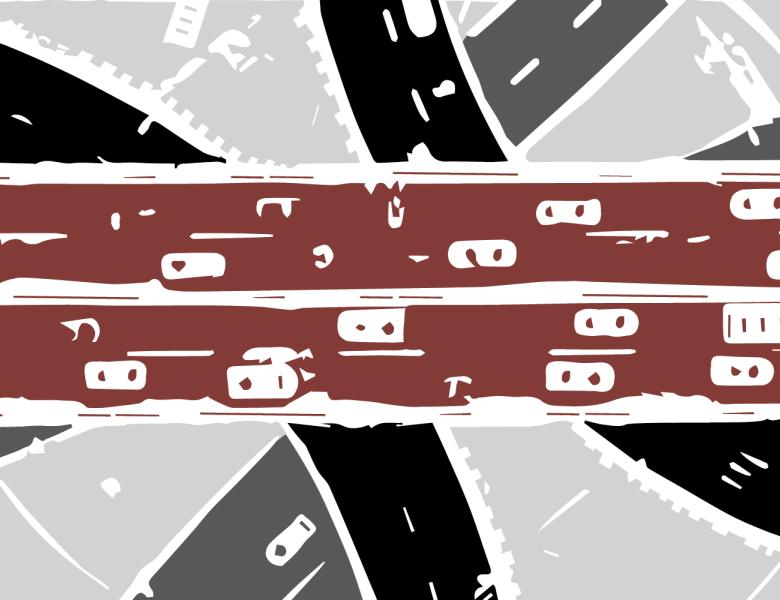
Abstract
The cost of sequencing has fallen to the point where prokaryotic genome sequencing is a vendor service; but while that sequence is easily obtained, determining what it actually does is expensive and somewhat risky. As a result enormous amounts of sequence data has been collected but can only be used for unsupervised learning; it cannot be mined for actionable hypotheses or leveraged for bioengineering. We know that bacteria have amazing metabolisms but we cannot begin to pinpoint where in their genomes they encode that behavior, so we cannot modify that behavior. We are building a platform for the automatic generation of massive-scale functional data to label bacterial genome sequences. With the data we will learn enough about bacterial function to enable the design of genomes with new and improved functions. We hope our efforts will yield true deep learning in genomics, which will form a solid basis for retrosynthesis — the engineering of cells to produce things that no extant cells can now make — at scale.