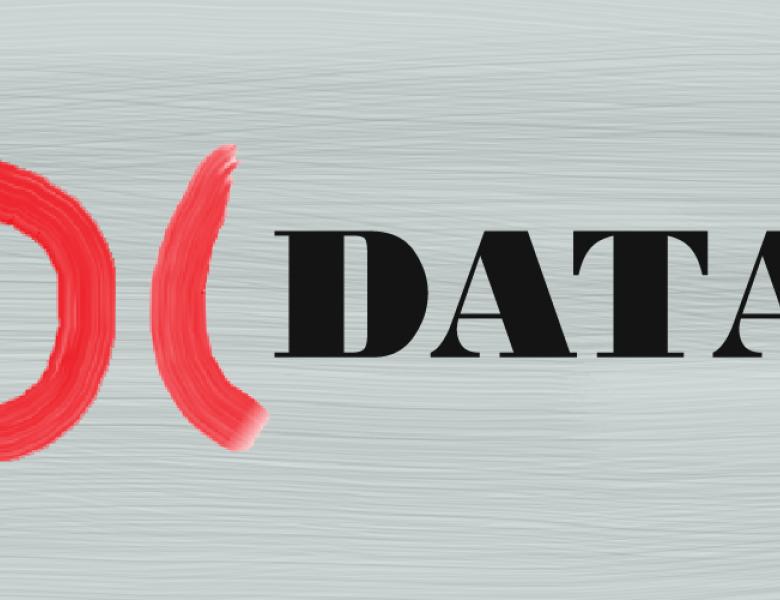
Abstract
Privacy definitions provide ways for trading-off the privacy of individuals in a statistical database for the utility of downstream analysis of the data. Recently we proposed Pufferfish a general framework for privacy definitions that provides data publishers with very fine grained control over the privacy definition. In this talk I will describe a sub-class of Pufferfish privacy definitions allows data publishers to extend differential privacy using a policy, which specifies (a) secrets, or information that must be kept secret, and (b) constraints that may be known about the data. Policies help data publishers explore a larger space of the privacy-utility tradeoffs while allowing composable mechanisms. I will formalize policies and present novel algorithms that can handle general specifications of sensitive information and certain count constraints. I will also briefly describe applications of policies that are being implemented in the US Census, and conclude with directions for future work.