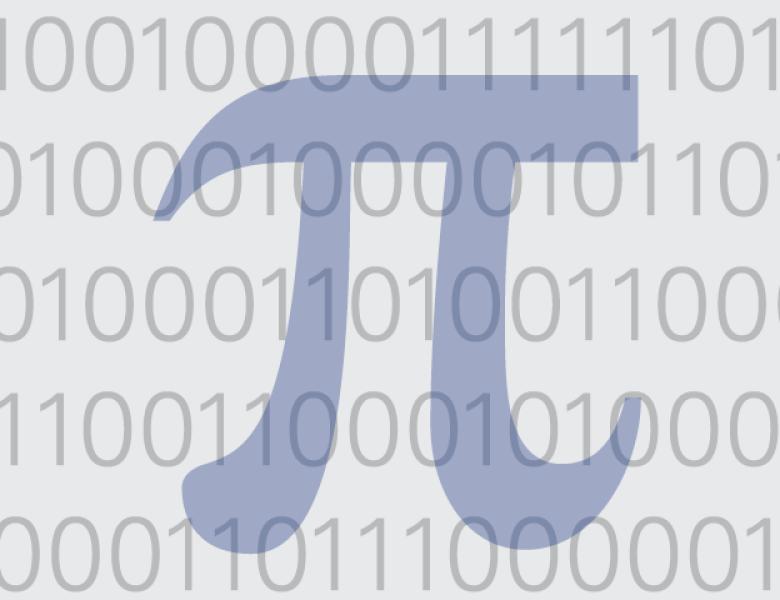
Abstract
Spectral gaps of matrices are related to many basic properties, like mixing times, expansion, isoperimetry and more. We will see a connection between spectral gaps and sign-rank. The sign-rank of a boolean matrix is the minimum dimension of real space in which the matrix can be realized as a point-halfspace incidence matrix. Sign-rank is deeply related to learning theory and communication complexity. We will see that regular matrices with large spectral gaps have high sign-rank; roughly speaking, this means that a matrix with large spectral gap can not be realized in low dimensional real space using halfspaces. This is another aspect in which matrices with a large spectral gap are pseudo-random. Based on work with Noga Alon and Shay Moran.