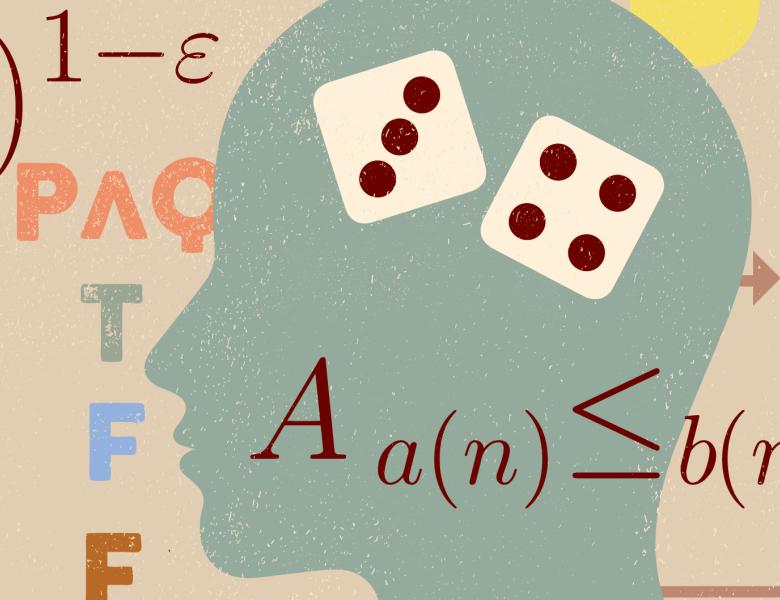
Abstract
Provenance-based explanations for query results have been the subject of extensive research since such explanations allow users to validate and justify the results of the query and deepen their knowledge about the data. However, when trying to apply these tools in complex settings, we face several challenges. In particular, raw provenance may not be easily understood by users due to its complexity and length. Furthermore, when the query encodes proprietary criteria and needs to remain confidential, provenance-based explanations may be detrimental to this privacy desideratum.
In this talk, I will present two works that address these challenges using provenance manipulation. I will begin by presenting a solution that generates provenance-based natural language explanations for query results. I will then proceed to discuss a recent work for ensuring that provenance-based explanations can be released while keeping a proprietary query hidden using a privacy model inspired by k-anonymity.