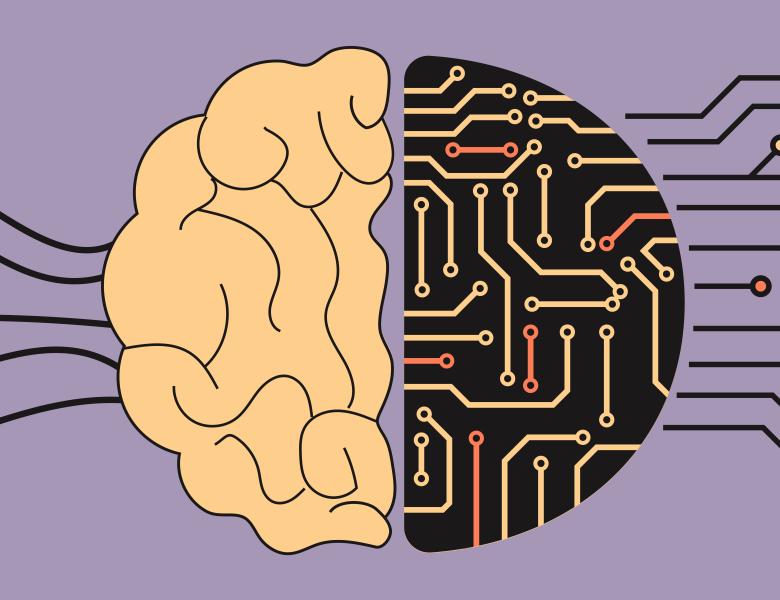
Abstract
While large language models (LLMs) seem to be ever-growing in size and in their prevalence in science and society, smaller "baby" language models (BabyLMs) are often better positioned to advance academic research. BabyLMs are well-suited to fundamental research in machine learning, linguistics, and cognitive science because they are faster and cheaper to train from scratch, and they are more plausible simulations of human learners. In this talk, I expand on this argument in my role as a founder of the BabyLM Challenge, a multi-year competition and workshop to improve data-efficiency, cognitive plausibility, and accessibility of LMs. I then discuss recent studies from my new lab at UCSD, training BabyLMs from scratch in controlled environments in order to advance far-reaching questions about language acquisition in humans and machines. We investigate the causes of the critical period for L2 learning, the inductive bias underlying typological tendencies in human language, and the distributional signatures of word learning trajectories in BabyLMs. While BabyLMs are sometimes tantalizingly human-like and other times spectacularly not so, in either case the exploration of a non-human language learner helps us better understand humans' place in the landscape of possible learners.