Mechanisms: Inside or In-Between?
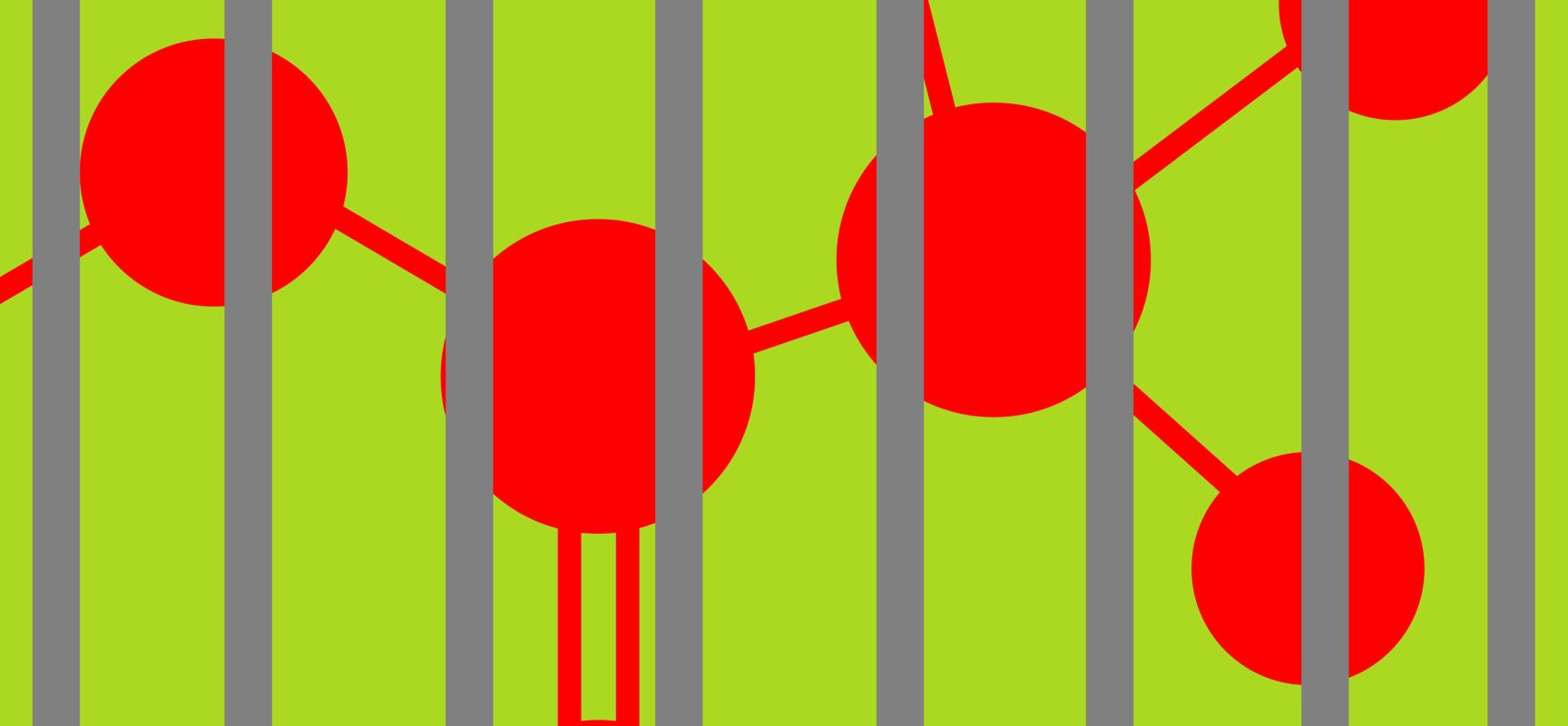
by Issa Kohler-Hausmann (Senior Law and Society Fellow, Spring 2022, Simons Institute)1
This work was made possible by the Simons Institute’s Causality program in the spring of 2022, where I was the Law and Society Fellow and had the opportunity to learn and discuss with a collection of brilliant scholars thinking about and working on causality and causal modeling. Special gratitude goes to Robin Dembroff, Maegan Fairchild, and Shamik Dasgupta, who participated in the April 2022 Theoretically Speaking event “Noncausal Dependence and Why It Matters for Causal Reasoning.”
Introduction
The term “mechanism” or “causal mechanism” is used in two possibly conflicting ways in causal inference literature. Sometimes “causal mechanism” is used to refer to the chain of causal relations that is unleashed between some stipulated triggering event (let’s call it X) and some outcome of interest (let’s call it Y). When people use the term in this sense, they mean “a causal process through which the effect of a treatment on an outcome comes about.”2 One could think of this use of the term as slowing down a movie about the causal process between the moment when X is unleashed and when Y obtains so that we can see more distinct frames capturing ever-finer-grained descriptions of prior events triggering subsequent events as they unfold over time. This is the in-between sense of “mechanism,” or, as Craver says, “causal betweenness.”3 An expansive methodological literature engages causal mechanisms in the in-between sense under the banner of mediation or indirect effects.4 When used in this way, a causal mechanism M lies in the middle of a causal pathway between X and Y: X → M → Y.
But there is a different sense of “mechanism” that refers to whatever it is about the triggering variable (let’s call it X again) that endows it with the causal powers it has. When people use the term in this inside sense, they mean to pick out the constituents of X, the parts and relations that compose it, or the grounds by virtue of which it obtains. Instead of slowing down the movie of a causal process unfolding over time, this use of “mechanism” calls for zooming into X at a particular slice in time.5
Causal models encode mechanisms in the inside sense insofar as denoting a variable (e.g., X) in the model entails denoting the stuff that builds the innards of X in the model.6 Designating variables expresses how the modeler has chosen to carve up states or events in the world. It entails expressing the boundaries of the relata (represented by variables) in the model, as variables marked out as, for example, X and M are taken to be distinct.7 But variable definition often leaves the innards of each relata designated by a variable name — what’s inside of X and M — opaque. And because most causal models we work with are not expressed in terms of fundamental entities (whatever those are — quarks and leptons, or something), variables are built out of or constituted by other things and connections between those things. The variables take the various states designated in the model because certain facts obtain. Inside causal mechanisms are the intravariable relata and relations that compose the variables and give them their distinctive causal powers.
Questions about mechanisms could be posed in one or the other sense of the term. For example, imagine you have a pile of pills and know with absolute certainty that each pill contains the identical chemical substance and dosage. Now imagine you conduct a randomized controlled trial with these pills to see whether ingesting these pills reduces reported headaches, and you document some average causal effect. Upon completion of the study, you might say: “We still do not know the causal mechanisms involved here.” There are simply two meanings to that query.
One meaning is that you do not know what physical processes in the body ingestion of the pill triggered — what physiological pathways ingestion of the substance brought about and unfolded over time such that headache pain was reduced. This version of the query asks about causal mechanisms in the in-between sense. Alternatively, you could mean that you do not know what was in the pill! That is, you have no idea what stuff did the triggering — you do not know the chemical compound that constituted the little pills you gave to your treated subjects.8 This version of the query asks about causal mechanisms in the inside sense, asking what facts obtained such that the thing designated as the cause occurred.
Sometimes people blur these two uses together.9 However, it is important to maintain this conceptual distinction because the relationship between mechanisms in the inside and in-between senses sets some limits on variable definition within a causal model. Specifically, if you posit some mechanism in the in-between sense in a causal model, then the state or event picked out by that mediator cannot be inside another variable.
An example
Start with a model expressed only in terms of abstract notation denoting a (indefinite article) variable, X, causing another variable, Y, positing two causal pathways from X to Y: a direct causal pathway from X → Y, and an indirect causal pathway from X → M → Y.
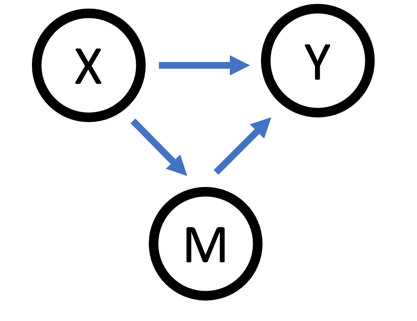
Now imagine you fill in the abstract notation that was just holding the place of actual relata that stand in these causal dependencies with specific events or states. Let the variable X designate ingestion of a pill consisting of 100 mg of acetylsalicylic acid (X = 1 if ingested the drug and X = 0 if otherwise), and let Y designate self-reported level of headache pain (Y = 1 if improved and Y = 0 if otherwise).
Notice this way of filling in variable X lumps together a series of events and states in X = 1 (ingesting a pill with 100 mg of acetylsalicylic acid) that could be more finely described. In this case, let’s assume you stipulate that X = 1 includes at least lifting the pill to one’s lips, swallowing the pill, the pill traversing the esophagus, and the substance that was swallowed being broken down to enter the bloodstream. Even so, it still leaves some ambiguity about the full list of necessary and sufficient truth conditions for the variable instantiations in this model — e.g., “X = 1” or “X = 0.” Said another way: it is not totally clear what facts must obtain in this world (or an alternative possible world) for it to be the case that the proposition expressed by the formal notation “X = 1” is true. Nothing in this brief description above requires, for example, that when X = 1, the person has to know or believe that the pill ingested contained 100 mg of acetylsalicylic acid. For the purposes of this example, let’s assume that this was intentional — you did not intend positive knowledge or belief regarding successful ingestion of 100 mg of acetylsalicylic acid to be included in X = 1.
M, version 1: placebo effect
Continuing with the formal causal model expressed in Figure 1, imagine you now define M as a variable picking out whether the subject knows or believes they have ingested 100 mg of acetylsalicylic acid (M = 0 if subject lacks knowledge of whether they ingested 100 mg of acetylsalicylic acid, M = 1 if subject knows or believes they ingested 100 mg of acetylsalicylic acid). The variables X and Y retain their definitions stipulated above. Perhaps you define M this way because you want to know what portion of headache reduction was caused by the mechanism of 100 mg of acetylsalicylic acid entering the bloodstream as opposed to the mechanism of knowing or believing one has ingested 100 mg of acetylsalicylic acid.
Your model expresses the assumption that every value each variable could take is compatible with every other variable taking any value it can take. That is, it must be possible for the truth conditions of X = 1 to obtain while, at the same time, the truth conditions for M = 0 to obtain, and so on for each variable and value. Again, by truth conditions I just mean what has to be the case in the world (or in an alternative possible world) such that the proposition expressed by the abstract notation and natural language is true — that what the model says holds under those variable conditions in fact holds.10
In this example, it must be possible for a person to ingest a pill containing 100 mg of acetylsalicylic acid (X = 1) while not knowing or believing that they have (M = 0). Most of us have no problem with the assumption that the truth conditions expressed by those two variable values can hold at once; it is the entire logic behind blind and double-blind randomized controlled trials. Therefore, in this example, the assumption required to make this a valid causal model — that each variable can take its range of possible values consistent with other variables taking their range of possible values — is not threatened by positing this particular variable as a mechanism in the in-between sense.
M, version 2: nanocage effect
But consider a different definition of the mediating variable M. The Google tells me that acetylsalicylic acid (aspirin) has the following molecular structure: C₉H₈O₄.
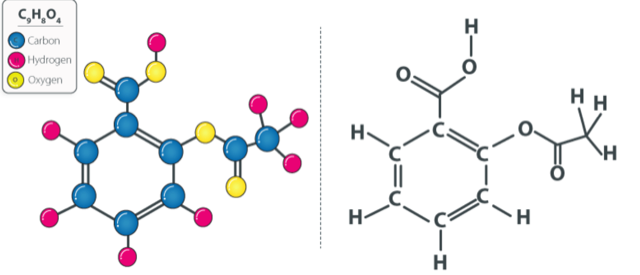
Now imagine you define M to be not-ingestion/ingestion {0,1} of that right-most portion of each aspirin molecule — the part that includes those three hydrogen and two oxygen atoms over there on the right. Perhaps you define M this way because you want to know what portion of headache reduction was caused by the ingestion of the remainder of the aspirin molecule net of its right-most portion. You propose building a nanocage around that portion of each aspirin molecule constituting the pill so that when the pill is swallowed, that right-most portion of each aspirin molecule cannot be broken down and absorbed into the bloodstream.11
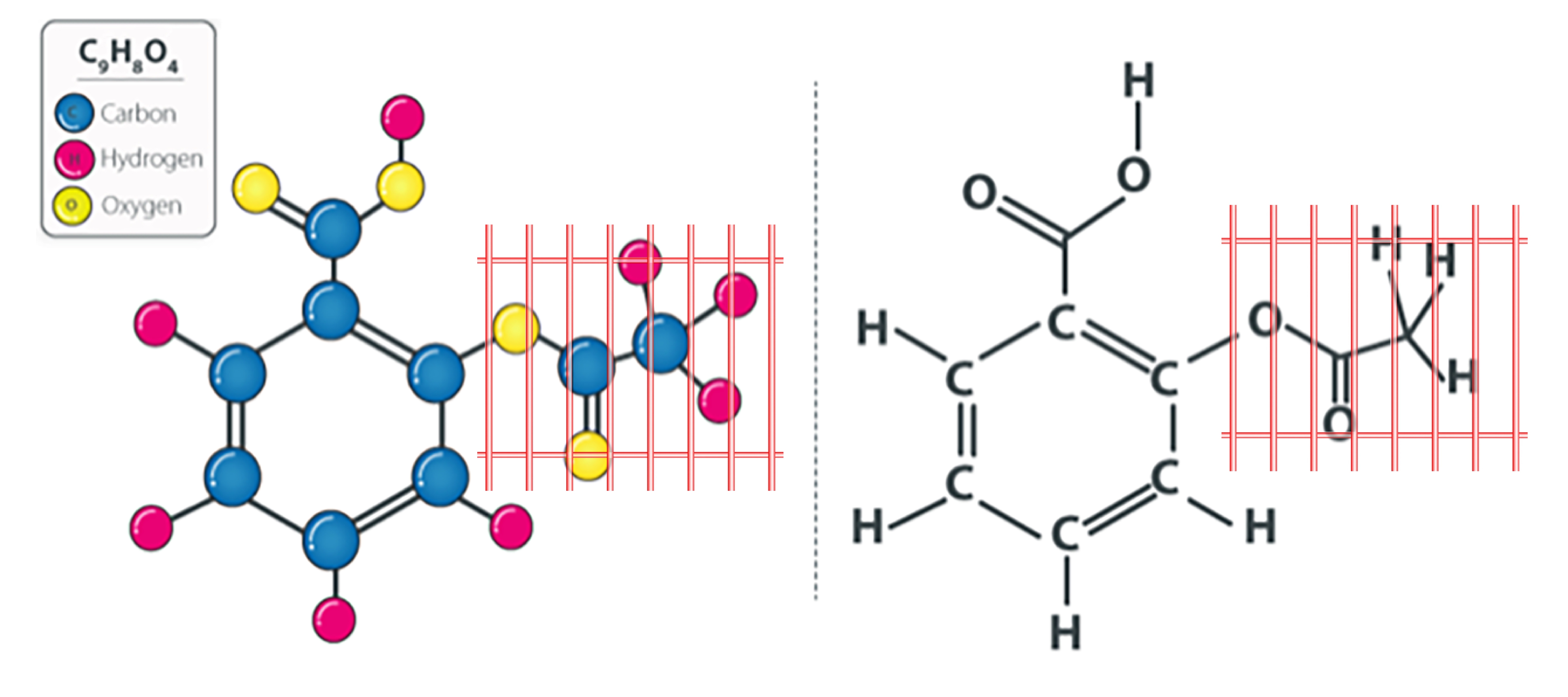
Remember that you have already defined X to be not-ingestion/ingestion of 100 mg of acetylsalicylic acid. And the truth conditions for X = 1 included (among other things) that the pill that was ingested contained a particular dosage of a particular chemical compound, and that such compound was broken down to enter the bloodstream. So here we have a problem with these variable definitions: the truth conditions for X = 1 (ingestion of 100 mg of acetylsalicylic acid) cannot hold when M = 0 (not-ingestion of the nanocaged portion of the molecule) because whatever your subjects are ingesting when M = 0, it is not acetylsalicylic acid. You have simply violated your own stipulative definition of the variables because part of M is inside of X (as you have defined X). That is, as the variables are defined in this model, for the truth conditions of X = 1 to obtain (the person ingests 100 mg of acetylsalicylic acid), the truth conditions for M = 1 must obtain (the person must ingest the entirety of the acetylsalicylic acid molecules).
Now you might say that it’s a free country, and you want to change your stipulative definition of what X = 1 entails. You change your definition to say that it entails just swallowing a pill containing 100 mg of acetylsalicylic acid, but not necessarily successfully ingesting the entirety of each acetylsalicylic acid molecule such that it is broken down to enter the bloodstream. Once you do so, there is no problem with X = 1 and M = 0 obtaining at the same time in this causal model. My response: I agree! Under this new definition, there is no problem (it matches the placebo effect example). I just want to point out why you felt compelled to change your definition of X: certain definitions of variables are taken off the table by how the other variables are defined because of what is inside them.12
Final thoughts
What is inside a variable is set by how we as modelers decide to carve up the world into variables in a model and the ontology of the resultant entities. But once we have done our carving and stipulated the meanings of our variables, we must check to see whether some of the things posited as mechanisms in the in-between sense are also necessary as mechanisms in the inside sense for other variables. If they are, we might have problems.
First, if there is a metaphysical reason that some combination of variable instantiations cannot happen at once, then the causal model cannot be used to define all the causal effects its formal relations propose. If, for example, there is no world in which X = 1 and at the same time M = 0, then we have carved up the variables in the model in a bad way.13 In the original nanocage effect example, certain potential outcomes and, therefore, certain causal effects are undefined (read: incoherent), such as Yi(X = 1, M = 0). Second, if we do not give sufficiently precise truth conditions for each variable instantiation, then the causal model does not communicate any clear propositions for evaluation and discussion. In the nanocage effect example, we could not debate whether there was a problem with the definitions of X and M in this model until we clearly understood what all was inside X (just swallowing the chemical compound? or swallowing and ingesting it?). Third, not knowing what is inside variables in a model can lead to misleading or false causal interpretations of counterfactuals specified by abstract notation. For example, the counterfactual contrast Yi(X = 1, M = 0) - Yi(X = 0, M = 0) cannot be interpreted to tell us anything about the effect of ingesting aspirin on headaches because the molecule represented in Figure 3 (which would be the substance ingested under conditions X = 1, M = 0) is not acetylsalicylic acid.14
Interrogating the ontology of the relata indicated by our model’s variables and specifying the truth conditions for each state or event picked out by each variable instantiation is not indulgent, bonbon-eating armchair philosophizing — to borrow my comrade Lily Hu’s colorful phrase. Rather, it is essential to the project of causal modeling and inference. In our running example, the variable X has the causal powers it does precisely because certain things are inside of it (causal mechanisms in the inside sense). If you take some of those things out by putting them into another variable (e.g., M, causal mechanism in the in-between sense), then X is no longer the same thing, and the resultant new entity would have different causal powers and propensities.
As a final note, the nanocage example explored here is just one instance where noncausal dependencies between explanatory relata raise vaguely specified “problems” with how a causal model has carved up the world into variables. The problems are obvious in this example because one variable is composed in part by another variable, so some settings of one variable require particular settings of another variable — e.g., if one ingests acetylsalicylic acid (X = 1), one must ingest all the components of acetylsalicylic acid (M = 1). However, there might also be problems even if the truth conditions for each variable value defined in the model could obtain at once. This can happen when the noncausal dependencies inside one variable (what builds or grounds the variable instantiations) are entangled or overlap in some way with the noncausal dependencies inside another variable.
Consider a causal model that seeks to identify the separate causal effects of the variables “winning the Sveriges Riksbank Prize in Economic Sciences in Memory of Alfred Nobel at time = 1” and “being influential in the field of economics at time = 1” on some outcome, say, “number of citations at time > 1.” It is certainly possible that, in some world, people could win the Nobel Memorial Prize in Economic Sciences whilst being unpopular and uninfluential in the field of economics. However, my guess is that we do not have much data on people who fit such a description because in our world being popular and influential in the field of economics seems to be among the (necessary, but not sufficient) grounds for winning the Nobel Memorial Prize in Economic Sciences. Therefore, if a causal model asks about the causal effect of, for example, winning the Nobel Memorial Prize in Economic Sciences while being unpopular and uninfluential in the field of economics, is it not clear whether such a model is asking about causal effects in a different world (e.g., a world where the grounds for winning such a prize are different than they are in our world) or about the causal effects of winning the prize and something else — the something that had to happen such that a person who did not meet the conditions for the award in our world was, nonetheless, awarded the prize (e.g., the winner blackmailed the judges, the judges went rogue).15 Here again, this question turns on what one intends to put inside the variables designated in the model — i.e., what has to be the case for the variable instantiations to be true.
If you do not like that example, consider the example used by Vera Hoffmann-Kolss of “being a sister” and “being a mother.” These variables are independently manipulable, and it is possible to have the truth conditions for each setting of one variable compatible with all settings of the other. However, having both in a causal model might be a problem because something that is inside one variable — the property of being coded female — is also inside the other. When are variables related in such a way that it is inappropriate to have them simultaneously in a causal model? I do not know. Folks, including Hoffmann-Kolss, have offered various proposals or tests for when noncausal dependencies between variables will — for lack of a better word — mess up the causal model.16 My point in this post is merely to raise this as an issue that is important to think about when we construct and interpret causal models.
-
Thank you to the Simons Institute for the Theory of Computing for a terrific semester full of learning and thoughtful exchanges. This work, like most of my current work, would not be possible without Lily Hu, my comrade in causal arms with whom much thinking on this topic was done and who graciously read a draft and provided invaluable feedback. I am also profoundly thankful for Frederick Eberhardt, who welcomed me to the Simons Institute program on Causality and with whom I have learned so much during many lunches and cocktails, and who also generously offered feedback that immensely improved this draft. I am grateful to Ned Hall for extended discussion and feedback, and also to Jamie Robins and Ilya Shpitser for engagement on this ongoing project. Sachin Holdheim provided tremendous research assistance and editing.↩︎
-
See, e.g., Kosuke Imai, Dustin Tingley & Teppei Yamamoto, Experimental Designs for Identifying Causal Mechanisms, 176 Journal of the Royal Statistical Society. Series A (Statistics in Society) 5, 5 (2013) (“We use the term causal mechanism to mean a causal process through which the effect of a treatment on an outcome comes about.”).↩︎
-
Carl F. Craver, Stuart Glennan & Mark Povich, Constitutive Relevance & Mutual Manipulability Revisited, 199 Synthese 8807, 8823 (2021). See also, Totte Harinen, Mutual Manipulability and Causal Inbetweenness, 195 Synthese 35 (2018).↩︎
-
See, e.g., James M. Robins, Thomas S. Richardson & Ilya Shpitser, An Interventionist Approach to Mediation Analysis, in Probabilistic and Causal Inference: The Works of Judea Pearl 713 (Hector Geffner, Rina Dechter & Joseph Y. Halpern, eds., 2022) (“Along these lines, in many contexts we may ask what ‘fraction’ of the (total) effect of A on Y may be attributed to a particular causal pathway.”).↩︎
-
See, e.g., Peter Hedström & Petri Ylikoski, Causal Mechanisms in the Social Sciences, 36 Annual Review of Sociology 49, 52 (2010) (“There is ambiguity in the use of the notion of a mechanism. Sometimes it is used to refer to a causal process that produces the effect of interest and sometimes to a representation of the crucial elements of such a process.”); Craver, Glennan & Povich, supra note 3, at 8810 (“To give a causal (Salmon says ‘etiological’) explanation for an explanandum event, E, one must determine which among the objects, processes, and events in E’s past are causally (and so explanatorily) relevant to its occurrence. In contrast, to give a constitutive explanation for E involves determining which objects, processes, and events constitute E.”).↩︎
-
These “building” relata might be other nonfundamental events or states and the relations between them, and those relations might be grounding, constitution, or other ways the variable is built. For an excellent discussion of building relations, see generally Karen Bennett, Making Things Up (2017).↩︎
-
See James Woodward, Interventionism and Causal Exclusion, 91 Philosophy and Phenomenological Research 303, 310 (2015) (“[I]t is assumed that we are dealing with variables [that] are ‘distinct’ in a way that allows them to be potential candidates for relata in causal relationships.”); see also Vera Hoffmann-Kolss, Interventionism and Non-Causal Dependence Relations: New Work for a Theory of Supervenience, 100 Australasian Journal of Philosophy 679 (2020). These authors offer different versions of what it takes for variables to be sufficiently “distinct” for mutual inclusion in a causal model.↩︎
-
Many careful readers will question how you can at once know for certain that all the different pills contain the same substance in identical dosage, but not know what that substance is. For purposes here, let’s just stipulate you know the pills are the same, but not what is in them. But this just shows that one cannot be completely ignorant of mechanisms in the inside sense and design an experiment; otherwise one would have no way of assigning the same treatment to different subjects.↩︎
-
See, e.g., Hedström & Ylikoski, supra note 5, at 52 (“There is an ambiguity in the use of the notion of a mechanism. … This should not be a cause for concern, however, because … [w]hen one makes a claim that a certain mechanism explains some real world events, one commits to the existence of the entities, properties, activities, and relations that the description of the mechanism refers to.”)↩︎
-
For a brilliant lengthy discussion of this and related issues, see generally Ned Hall, Structural Equations and Causation, 132 Philosophical Studies 109 (2007).↩︎
-
Thank you to Jamie Robins for this evocative idea.↩︎
-
Here I use “inside” to refer to any kind of noncausal dependence (e.g., composition, constitution, grounding). In this short post, I do not defend any particular test for whether something is inside of X such that putting it inside of M raises problems, but do note that such concerns extend beyond just mathematical deterministic relations, identity, or full composition.↩︎
-
Words like “problem” and “bad” are about as specific as I am going to get in this post.↩︎
-
For a related discussion that seeks to specify the meaning of a causal effect defined by holding constant a mediator variable while toggling its upstream parent by redefining the causal variable into its constituent components, see generally Robins, Richardson & Shpitser, supra note 4.↩︎
-
See Hu & Kohler-Hausmann.↩︎
-
Hoffmann-Kolss, supra note 7, argues that variables that share overlapping minimal supervenience bases should not be included in a causal model together.↩︎