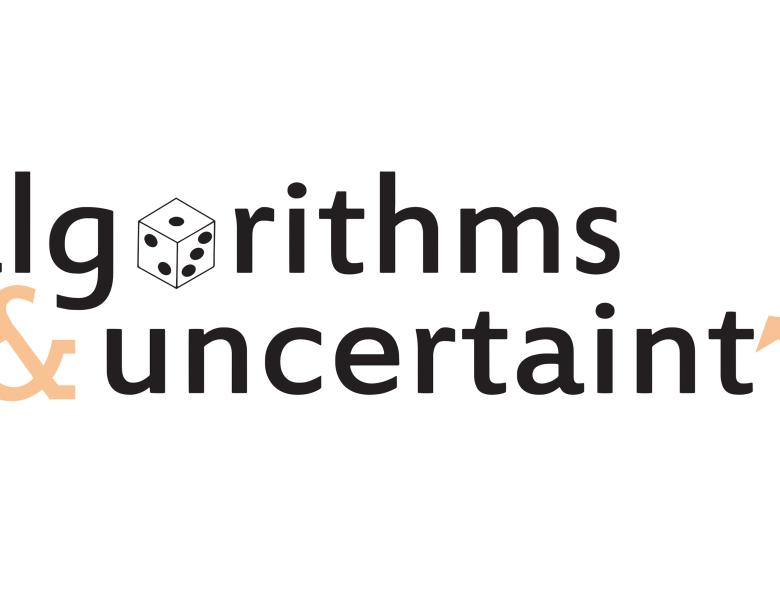
Stochastic Geometric Optimization Problems and Coresets
We consider the standard stochastic geometry model in which the existence or the location of each point may be stochastic. We show there exists constant-size kernel coreset (a kernel can approximate either the expected value or the distribution of the width of the point set in every direction) and we can construct such kernel coresets in nearly linear time. We show its applications to several function extent problems, tracking moving points, and shape fitting problems. We also study two important geometric optimization problems, the k-center problem and the j-flat-center problem, over stochastic data points in Euclidean spaces. We provide the first PTAS for both problems. I will talk about several concepts that are useful, such as Minkovski sum, coresets, geometric duality, Tukey depth, VC convergence bound.
Joint work with Lingxiao Huang, Jeff Phillips, Haitao Wang.
All scheduled dates:
Upcoming
No Upcoming activities yet