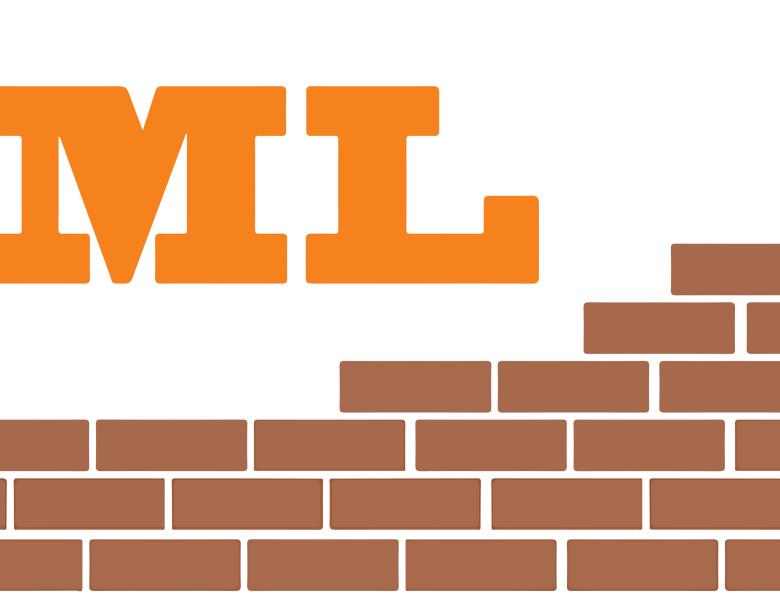
Sequential Information Maximization
Optimal information gathering using uncertain observations is a central challenge in many disciplines of machine learning such as Bayesian experimental design, automated diagnosis, active learning, and decision making. A widely used method is to perform sequential observation selection, where the choice of the next observation depends on the history seen thus far. Despite the importance and widespread use in applications, little is known about the theoretical properties of sequential observation selection policies in the presence of noise. In particular, a long-open direction has been to analyse the persistent-noise setting that is arguably more relevant in practical applications. In this talk, we will present a new framework to capture the role of noise, and explain how it leads to the first rigorous analysis of the famous information-gain policy. We will then consider more general information gathering settings and provide new efficient algorithms, with provable guarantees, to deal with noisy observations.
Joint work with Yuxin Chen, Andreas Krause, and Amin Karbasi
All scheduled dates:
Upcoming
No Upcoming activities yet