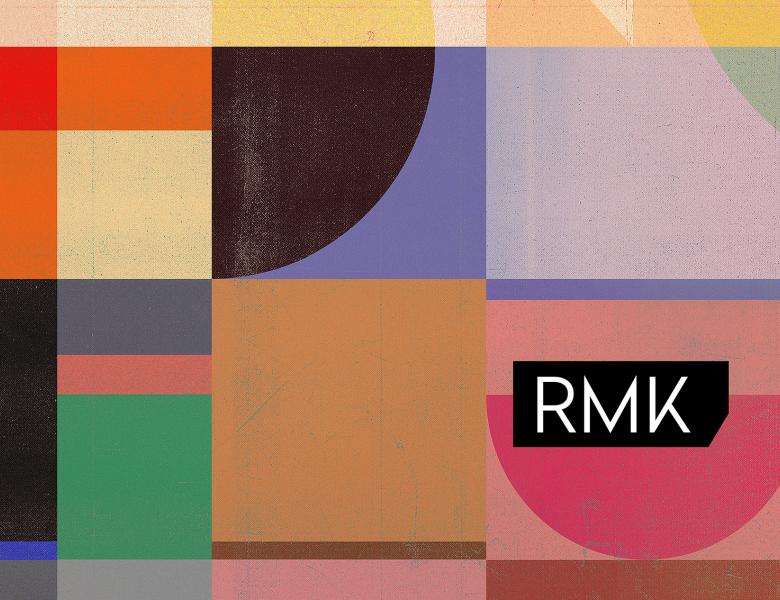
Abstract: Graphons and graphexes are limits of graphs that allow us to model and estimate properties of large-scale networks. Graphons are by now widely used in theory and applications; indeed, they are the topic of much of the Simons Institute’s semester program on Graph Limits and Processes on Networks: From Epidemics to Misinformation. For dense graphs, there are many equivalent ways to define the limit, some more global and others more local in nature. For sparse graphs of unbounded degree, there are two alternative theories for finding the limits — a probabilistic approach that leads to bounded graphons over probability spaces, and a statistical approach leading to graphexes over sigma-finite measure spaces. The former is in many ways the extension of the “global approach” for dense graphs. For some time, it was believed that there was no natural way to extend the local notions to sparse graphs. However, the statistical approach finally emerged, and that is what this talk will focus on. After a very brief review of the theory for dense graphs and the probabilistic approach for sparse graphs, this talk will formulate the statistical approach. It will recast limits of dense graphs in terms of exchangeability and the Aldous-Hoover theorem, and then generalize this to obtain sparse graphons and graphexes as limits of subgraph samples from sparse graph sequences. This will provide a dual view of sparse graph limits as processes and random measures, an approach that allows a generalization of many of the well-known results and techniques for dense graph sequences. This statistical approach is better suited to describing the evolution of large sparse networks.
Jennifer Chayes is associate provost of UC Berkeley’s Division of Computing, Data Science, and Society, and dean of its School of Information. She is a professor of EECS, Information, Mathematics, and Statistics. For 23 years, she was at Microsoft, most recently as a technical fellow, where she cofounded and led three interdisciplinary labs in Cambridge, Massachusetts; New York City; and Montreal. She is a member of the National Academy of Sciences and the American Academy of Arts and Sciences. Chayes has received numerous awards and honors, including the 2015 John von Neumann Prize of the Society for Industrial and Applied Mathematics (the highest honor of SIAM) and honorary doctorates from Bard College and Leiden University. Chayes is one of the inventors of graphons, widely used in machine learning of networks. Her recent work also includes applications of machine learning to cancer immunotherapy, ethical decision‐making, and climate and sustainability.
=========================================================
The Richard M. Karp Distinguished Lectures were created in Fall 2019 to celebrate the role of Simons Institute Founding Director Dick Karp in establishing the field of theoretical computer science, formulating its central problems, and contributing stunning results in the areas of computational complexity and algorithms. Formerly known as the Simons Institute Open Lectures, the series features visionary leaders in the field of theoretical computer science, and is geared toward a broad scientific audience. Light refreshments will be available prior to the start of the lecture. This lecture will be viewable thereafter on this page and on our YouTube channel.
Please note: the Simons Institute regularly captures photos and video of activity around the Institute for use in videos, publications, and promotional materials.
All scheduled dates:
Upcoming
No Upcoming activities yet