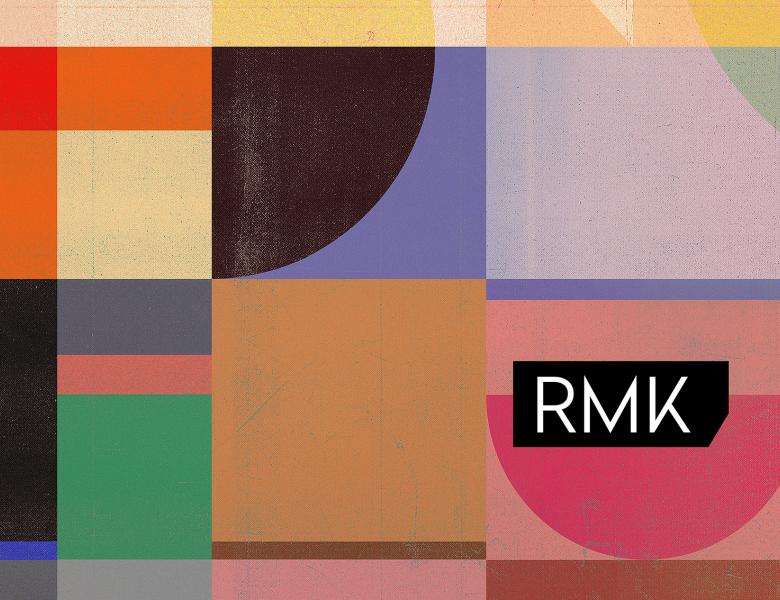
Massive data collection holds the promise of a better understanding of complex phenomena and, ultimately, of better decisions. An exciting opportunity in this regard stems from the growing availability of perturbation/intervention data (for example, in genomics, advertising, education, etc.). To obtain mechanistic insights from such data, a major challenge is the development of a framework that integrates observational and interventional data and allows causal transportability — i.e., predicting the effect of yet-unseen interventions or transporting the effect of interventions observed in one context to another. I will propose an autoencoder framework for this problem. In particular, I will characterize the implicit bias of overparameterized autoencoders and show how this links to causal transportability and can be applied for drug repurposing in the current COVID-19 crisis.
Caroline Uhler is an associate professor at MIT in EECS and the Institute for Data, Systems, and Society. Her research focuses on graphical models, causal inference, and applications to biology. Uhler’s main research is in mathematical statistics and its applications to computational biology. She is particularly interested in the area of algebraic statistics, which exploits the use of algebraic techniques to develop new paradigms and algorithms for data analysis. In her research, she uses methods from algebraic geometry, computational algebra, and combinatorics to study statistical theory problems, such as parameter estimation and identification in graphical models. Besides her theoretical interests, she also works on developing mathematical and statistical tools for applications to computational biology.
Light refreshments will be provided before the lecture. Due to current health directives the refreshments will be set up outside the building. There will be signs to direct you. Food and drinks are not permitted in Calvin Lab at this time,
If you require accommodation for communication, please contact our Access Coordinator at simonsevents@berkeley.edu with as much advance notice as possible.
Please note: the Simons Institute regularly captures photos and video of activity around the Institute for use in videos, publications, and promotional materials.
All scheduled dates:
Upcoming
No Upcoming activities yet