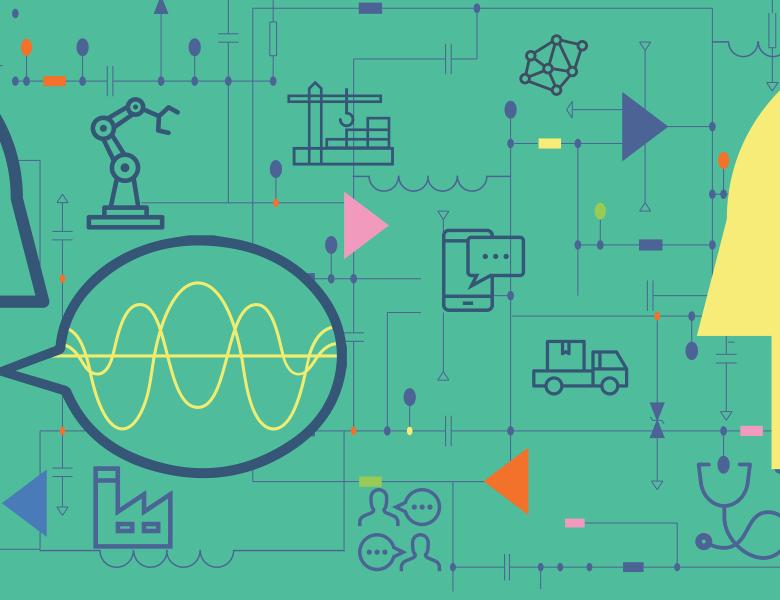
Episodic reinforcement learning and contextual bandits are two widely studied sequential decision-making problems. Episodic reinforcement learning generalizes contextual bandits and is often perceived to be more difficult due to long planning horizon and unknown state-dependent transitions. The current paper shows that the long planning horizon and the unknown state-dependent transitions (at most) pose little additional difficulty on sample complexity. We consider the episodic reinforcement learning with S states, A actions, planning horizon H, total reward bounded by 1, and the agent plays for K episodes. We propose a new algorithm, \textbf{M}onotonic \textbf{V}alue \textbf{P}ropagation (MVP), which relies on a new Bernstein-type bonus. The new bonus only requires tweaking the \emph{constants} to ensure optimism and thus is significantly simpler than existing bonus constructions. We show MVP enjoys an O((\sqrt{SAK}+S^2A)polylog(SAHK)) regret, approaching the Ω(\sqrt{SAK}) lower bound of \emph{contextual bandits}. Notably, this result 1) \emph{exponentially} improves the state-of-the-art polynomial-time algorithms by Dann et al. [2019], Zanette et al. [2019] and Zhang et al. [2020] in terms of the dependency on H, and 2) \emph{exponentially} improves the running time in [Wang et al. 2020] and significantly improves the dependency on S, A and K in sample complexity.
Authors: Zihan Zhang, Xiangyang Ji, Simon S. Du
All scheduled dates:
Upcoming
No Upcoming activities yet