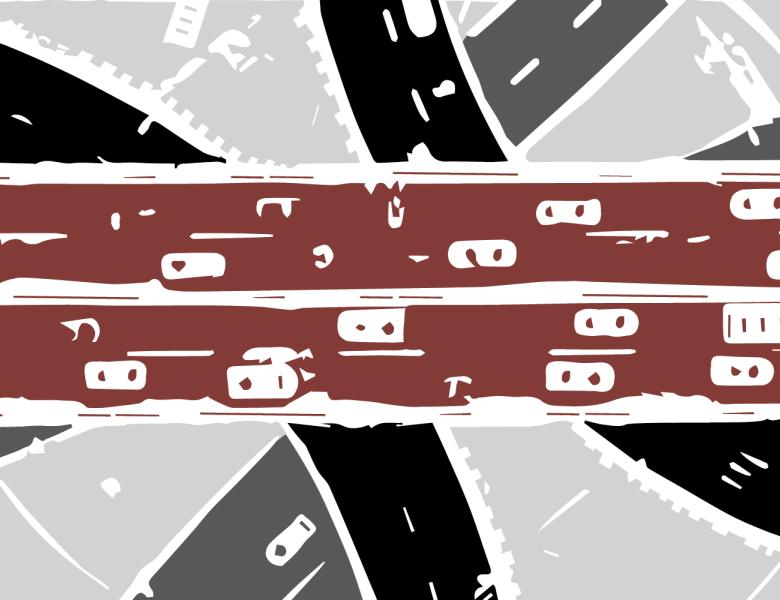
Online Learning in Games
The archetypal setting of online learning can be summarized as follows: at each stage of a repeated decision process, an agent selects an action from some set X (continuous or discrete), they obtain a reward based on an a priori unknown payoff function, and the process repeats. The most widely used optimization criterion in this framework is that of regret minimization - i.e., minimizing the aggregate payoff gap between the chosen action and the best fixed action in hindsight. However, in a multi-agent environment where an agent's reward is determined by that of all other agents via a fixed mechanism (the game), finer criteria apply, chief among them being that of convergence to a Nash equilibrium. In this talk, I will survey some recent equilibrium convergence and non-convergence results, in both finite and continuous games.
Anyone who would like to give one of the weekly seminars on the RTDM program can fill in the survey at https://goo.gl/forms/Li5jQ0jm01DeYZVC3.
All scheduled dates:
Upcoming
No Upcoming activities yet