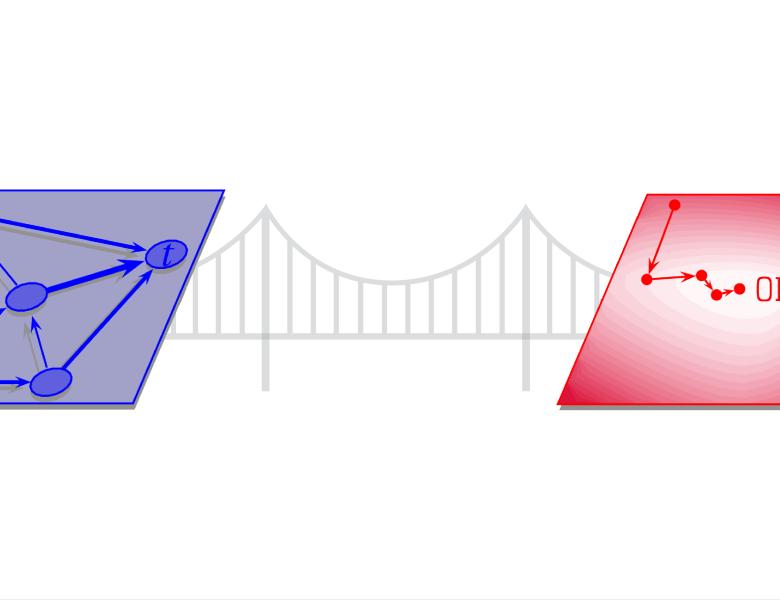
The Statistical Foundations of Learning to Control
Given the dramatic successes in machine learning and reinforcement learning (RL) over the past half decade, there has been a resurgence of interest in applying these techniques to continuous control problems in robotics, self-driving cars, and unmanned aerial vehicles. Though such applications appear to be straightforward generalizations of standard RL, few fundamental baselines have been established prescribing how well one must know a system in order to control it. In this talk, I will discuss the general paradigm for RL and how it is related to more classical concepts in control. I will then describe a contemporary view merging techniques from statistical learning theory and robust control to derive baselines for these continuous control problems. I will explore several examples that balance perception and action, and demonstrate finite sample tradeoffs between estimation and control performance. I will close by listing several exciting open problems that must be solved before we can build robust, safe learning systems that interact with an uncertain physical environment.
All scheduled dates:
Upcoming
No Upcoming activities yet