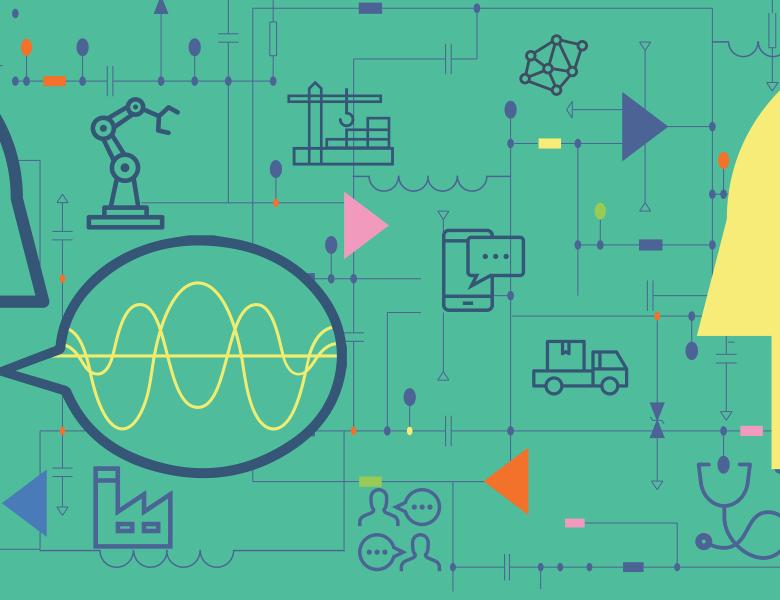
The Off-Policy Evaluation aims at estimating the performance of target policy π using offline data rolled in by a logging policy μ. Intensive studies have been conducted and the recent marginalized importance sampling (MIS) achieves the sample efficiency for OPE. However, it is rarely known if uniform convergence guarantees in OPE can be obtained efficiently. In this paper, we consider this new question and reveal the comprehensive relationship between OPE and offline learning for the first time. For the global policy class, by using the fully model-based OPE estimator, our best result is able to achieve ϵ-uniform convergence with complexity O˜(H^3⋅min(S,H)/d_mϵ^2), where d_m is an instance-dependent quantity decided by μ. This result is only one factor away from our uniform convergence lower bound up to a logarithmic factor. For the local policy class, ϵ-uniform convergence is achieved with the optimal complexity O˜(H^3/d_mϵ^2) in the off-policy setting. This result complements the work of sparse model-based planning (Agarwal et al. 2019) with generative model. Lastly, one interesting corollary of our intermediate result implies a refined analysis over simulation lemma.
Authors: Ming Yin, Yu Bai, Yu-Xiang Wang
All scheduled dates:
Upcoming
No Upcoming activities yet