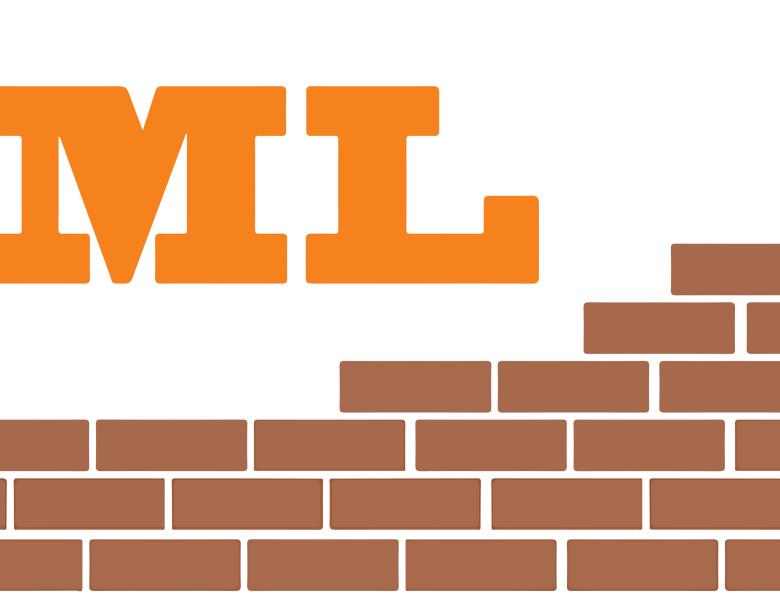
Description
Greed is Good If We Add A Bit of Randomness
It is known that greedy methods perform well for maximizing monotone submodular functions. At the same time, such methods perform poorly in the face of non-monotonicity. In this talk, I show--arguably, surprisingly-- that invoking the classical greedy algorithm O(\sqrt{k})-times leads to the (currently) fastest deterministic algorithm for maximizing a general submodular function subject to k-independent system constraints. This algorithm achieves (1 + O(1/\sqrt{k}))k approximation using O(nr\sqrt{k}) function evaluations (here, n and r denote the size of the ground set and the maximum size of a feasible solution, respectively). I then show that by a careful random sampling procedure, we can run the greedy algorithm only once and obtain the (currently) fastest randomized algorithm for maximizing a submodular function subject to k-extendible system constraints (a subclass of k-independent system constrains). This randomized algorithm achieves (k + 3)-approximation with only O(nr/k) function evaluations. Finally, I derive an almost matching lower bound, and show that no polynomial time algorithm can have an approximation ratio smaller than (k + 1/2 - \epsilon). No background in submodularity is needed. I will define all the important concepts during the talk. This is joint work with my student Chris Harshaw (Yale) and Moran Feldman (Open University of Israel).
All scheduled dates:
Upcoming
No Upcoming activities yet