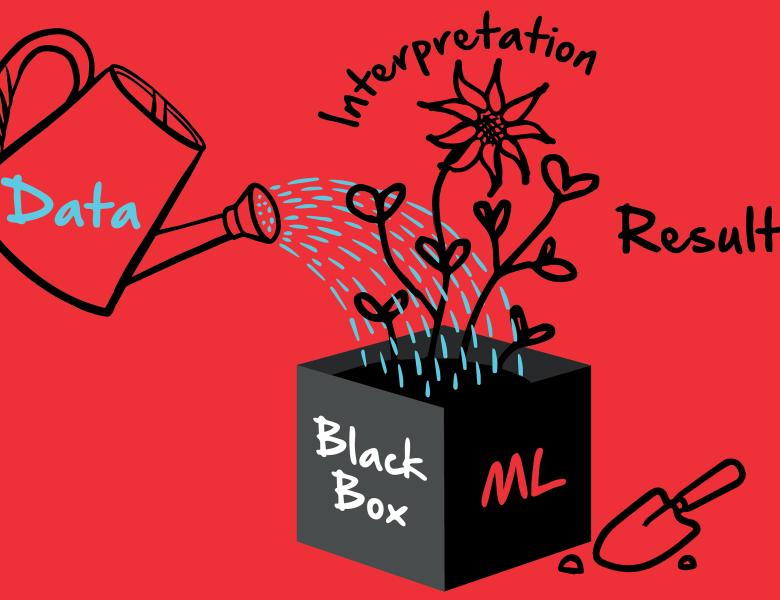
Note: The event time listed is set to Pacific Time.
I'll talk about several models in which a learner has access to both labeled data as well as some additional information that can be thought of as simple explanations for some of the labels.
1. Predictive feature feedback The learner starts with an unlabeled data set, and is allowed to query the labels of some of the points. With each label, it also receives the identity of a feature that is weakly predictive of that label.
2. Discriminative feature feedback This is an online setting in which, at each point in time, a new unlabeled instance arrives. The learner predicts the label and also supplies a previously-seen example that it deems "similar". It then receives the correct label, and in the case of an error, the identity of a relevant feature that separates the current instance from the supplied previous example.
3. Teaching We consider an interactive model of teaching in which the teacher probes the learner to see what it has understood, before supplying a new teaching example.
4. Learning from weak rules This is a setting in which the learner has a small amount of labeled data as well as a collection of "rules-of-thumb" of arbitrary accuracy. How can these rules be combined to get a good classifier?
In these models, the additional information makes it possible to learn more efficiently than in the classic supervised learning framework and to output a classifier that accompanies its predictions with simple explanations.
All scheduled dates:
Upcoming
No Upcoming activities yet