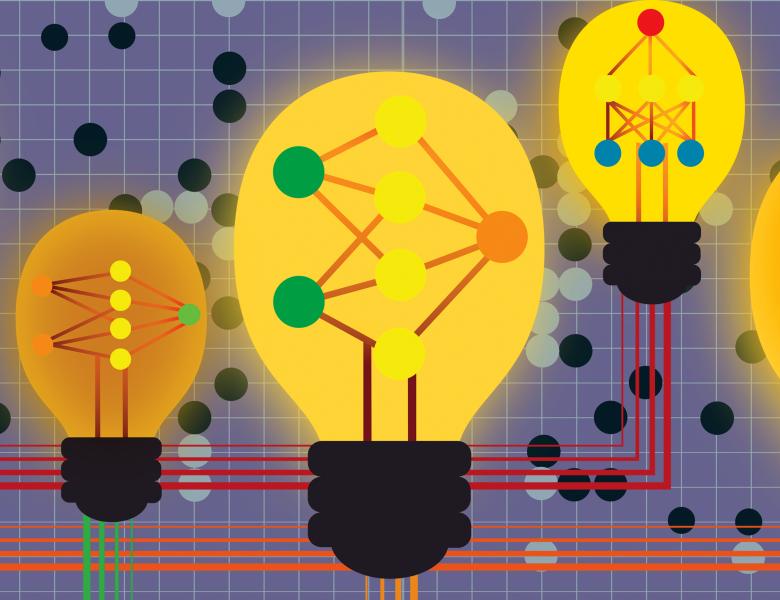
Title: A fundmental tension between no-regret and last-iterate convergence under realization-based feedback
Abstract: We study the limiting behavior of the mixed strategies that result from optimal no-regret learning strategies in a repeated game setting where the stage game is a 2 × 2 zero-sum game or competitive game (i.e. the Nash equilibrium is unique, strictly mixed, and trivially computable). We consider optimal no-regret algorithms that are approximately mean-based and monotonic in their argument. We show that for any such algorithm, the limiting mixed strategies of the players cannot converge almost surely to any Nash or correlated equilibrium. This negative result is also shown to hold under a broad relaxation of these assumptions, including popular variants of Online-Mirror-Descent with optimism or data-adaptive step-sizes, and parameter-free online algorithms. Our results identify the inherent stochasticity in players’ realizations as a critical factor underlying this divergence, and demonstrate a crucial difference in outcomes between using the opponent’s mixtures and realizations to make updates.
We conclude with a brief description of some open problems in last-iterate and time-averaged convergence under realization-based feedback.
This talk reflects joint work with Soham Phade (Salesforce) and Anant Sahai (UC Berkeley), and is based on the paper: https://arxiv.org/pdf/2012.
Bio: Vidya Muthukumar is an Assistant Professor in the Schools of Electrical and Computer Engineering and Industrial and Systems Engineering at Georgia Institute of Technology. Her broad interests are in game theory, online and statistical learning. She is particularly interested in designing learning algorithms that provably adapt in strategic environments, the intersection of algorithmic game theory and reinforcement learning, and the theory of overparameterized machine learning.
Vidya received the PhD degree in Electrical Engineering and Computer Sciences from University of California, Berkeley. She is the recipient of the Adobe Data Science Research Award, the Simons-Berkeley Research Fellowship (for the Fall 2020 program on "Theory of Reinforcement Learning"), and a Georgia Tech Class of 1969 Teaching Fellowship for the academic year 2021-2022.
All scheduled dates:
Upcoming
No Upcoming activities yet