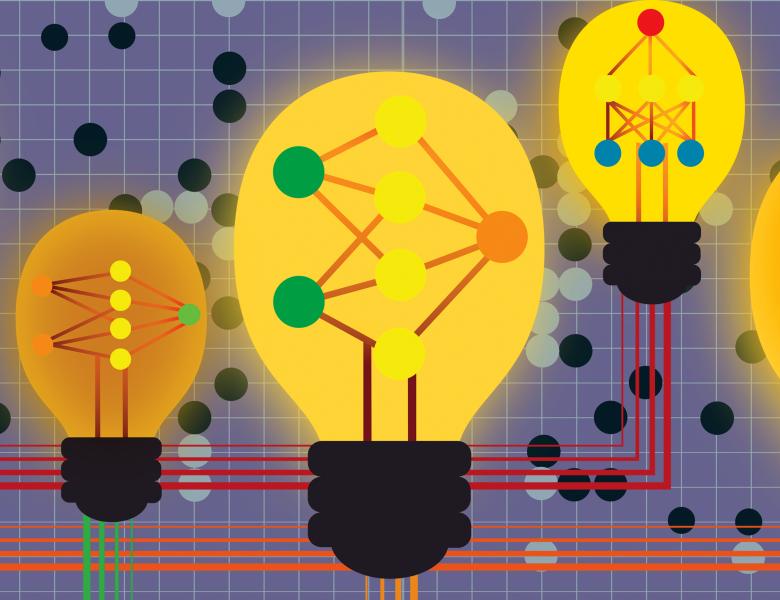
Title: Near-Optimal Learning of Extensive-Form Games with Imperfect Information
Abstract: Imperfect Information Games such as Poker constitute an important challenge for modern artificial intelligence. In this talk we consider the problem of learning Imperfect-Information Extensive-Form Games (IIEFGs), a celebrated formulation for games involving both imperfect information and sequential play. IIEFGs is a generalization of normal-form games, and is related to (but poses quite different challenges from) Markov Games.In the first part of the talk, we will review the definition and basic properties of IIEFGs, and go through existing algorithms such as Online Mirror Descent and Counterfactual Regret Minimization. In the second part of the talk, we present our new result---A first line of algorithms that require only $\widetilde{\mathcal{O}}((XA+
Bio: Yu Bai is currently a Senior Research Scientist at Salesforce AI Research. Prior to joining Salesforce, Yu completed his PhD in Statistics at Stanford University. Yu’s research interest lies broadly in machine learning, with recent focus on the theoretical foundations of reinforcement learning and games, deep learning, and uncertainty quantification.
List of related paper: https://arxiv.org/abs/
All scheduled dates:
Upcoming
No Upcoming activities yet