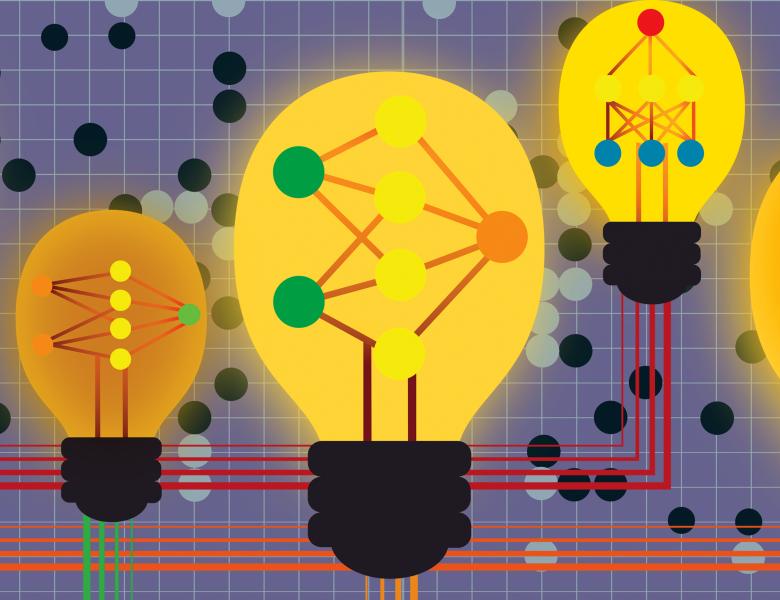
Title: Continuous Dynamics: Failures & Successes in Saddle Point Problems (in person)
Abstract: There will be two parts, in the first one I will present the work by Hsieh, Mertikopoulos and Cevher from ICML 2021, and in the second part I will present our joint work with Manolis Zampetakis and Michael I. Jordan -- see their abstracts below, respectively.
Compared to minimization, the min-max optimization in machine learning applications is considerably more convoluted because of the existence of cycles and similar phenomena. Such oscillatory behaviors are well-understood in the convex-concave regime, and many algorithms are known to overcome them. In this paper, we go beyond this basic setting and characterize the convergence properties of many popular methods in solving non-convex/non-concave problems. In particular, we show that a wide class of state-of-the-art schemes and heuristics may converge with arbitrarily high probability to attractors that are in no way min-max optimal or even stationary. Our work thus points out a potential pitfall among many existing theoretical frameworks, and we corroborate our theoretical claims by explicitly showcasing spurious attractors in simple two-dimensional problems.
Several widely-used first-order saddle point optimization methods yield an identical continuous-time ordinary differential equation (ODE) to that of the Gradient Descent Ascent (GDA) method when derived naively. However, their convergence properties are very different even on simple bilinear games. We use a technique from fluid dynamics called High-Resolution Differential Equations (HRDEs) to design ODEs of several saddle point optimization methods. For bilinear games, the convergence properties of the derived HRDEs correspond to that of the starting discrete methods. Using these techniques, we show that the HRDE of Optimistic Gradient Descent Ascent (OGDA) has last-iterate convergence for general monotone variational inequalities. To our knowledge, this is the first proof in continuous-time for monotone variational inequalities. Moreover, we provide the rates for the best-iterate convergence of the OGDA method, relying solely on the first-order smoothness of the monotone operator.
Bio: Tatjana Chavdarova is a Postdoctoral Researcher at the Department of Electrical Engineering and Computer Science (EECS) at the University of California at Berkeley, working with Michael Jordan. Prior to that she was postdoc at the Machine Learning and Optimization (MLO) lab at EPFL, working with Martin Jaggi, and she obtained her Ph.D. from EPFL, and Idiap, supervised by François Fleuret. She is primarily interested in the intersection of game theory and machine learning, but also generalization, robustness, and generative modeling. For further details, her website is https://chavdarova.github.
Paper connected with this talk:
1st part: https://arxiv.org/pdf/
2nd part: https://arxiv.org/pdf/
All scheduled dates:
Upcoming
No Upcoming activities yet