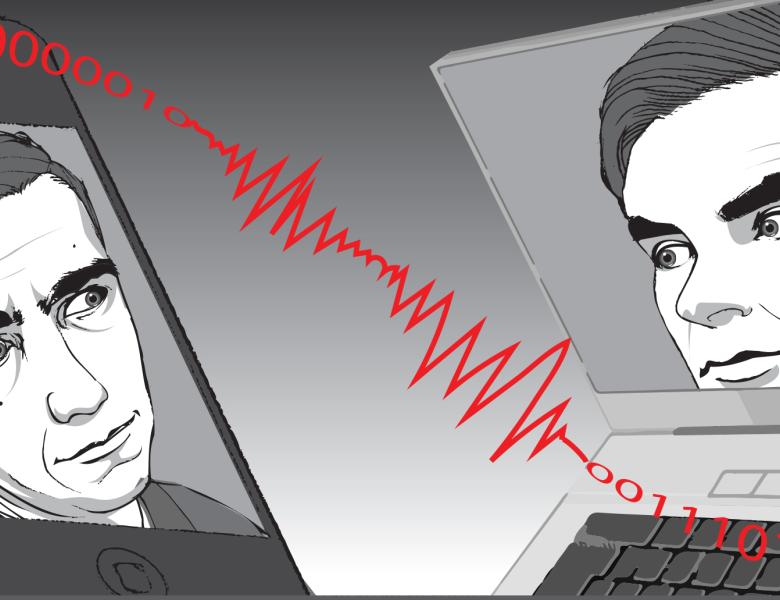
Algorithmic design via efficient data representations
The growing scale of data demands novel algorithmic design frameworks that are able to handle modern datasets. In this talk, I will describe how such frameworks emerge from the methods of efficient data representations. The first illustration will be the Nearest Neighbor Search (NNS) problem --- an ubiquitous massive datasets problem that is of key importance in machine learning and other areas. Its goal is to preprocess a dataset of objects (e.g., images), so that later, given a new query object, one can efficiently return the object most similar to the query. Efficient solutions may be achieved via Locality Sensitive Hashing (LSH), a data representation method that has seen a lot of success in both theory and practice.
I will present the best possible LSH-based algorithm for NNS under the Euclidean distance. Then, I will show a new method that, for the first time, provably outperforms the LSH-based algorithms.
Taking a broader perspective, I will describe other examples where the lens of "efficient data representation" leads to novel fast algorithms. These examples include a classic dynamic programming problem (edit distance), a Linear Program problem (Earth-mover distance), and an algorithmic framework for parallel models of computation (such as MapReduce).