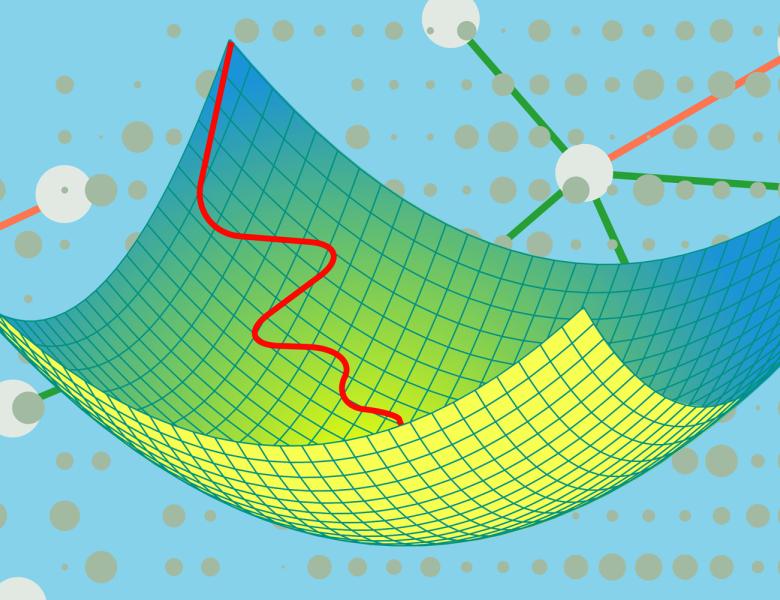
All scheduled dates:
Upcoming
No Upcoming activities yet
Past
TITLE: Interior Point Methods with a Gradient Oracle
Gradient methods are popular optimization primitives, since they rely
entirely on first-order information, which is usually easy to obtain.
Nevertheless, many interesting problems, such as those appearing in
conic optimization involve hard constraints, which require the use of
more sophisticated techniques (e.g. cutting planes or interior point
methods). These typically involve exploiting higher order information
about the objective or the geometry of the domain, such as the Hessian
matrix.
In this whiteboard talk, I will show how to overcome this difficulty,
by efficiently executing an interior point method which does not have
direct access to second order derivatives, and is solely based on
gradient information.
The key ingredient of this method is a beautiful idea of
[Dunagan-Harvey '07], where it was shown how to solve linear sytems of
equations while simultaneously constructing a preconditioner. This
approach can be leveraged in the context of interior point methods,
where one needs to solve a sequence of linear systems with a slowly
changing matrix.
As a consequence, we can compute high precision solutions to linear
optimization problems over (reasonably well-conditioned) n-dimensional
convex bodies using O~(n) gradient queries to an appropriate barrier
function, plus an extra cubic runtime overhead.
TITLE: Locally Uniform Hashing
Hashing is a common technique used in data processing, with a strong impact on the time and resources spent on computation. Hashing also affects the applicability of theoretical results that often assume access to (unrealistic) uniform/fully-random hash functions. In this paper, we are concerned with designing hash functions that are practical and come with strong theoretical guarantees on their performance.
To this end, we present tornado tabulation hashing, which is simple, fast, and exhibits a certain full, local randomness property that provably makes diverse algorithms perform almost as if (abstract) fully-random hashing was used. For example, this includes classic linear probing, the widely used HyperLogLog algorithm of Flajolet, Fusy, Gandouet, Meunier [AOFA'97] for counting distinct elements, and the one-permutation hashing of Li, Owen, and Zhang [NIPS'12] for large-scale machine learning. We also provide a very efficient solution for the classical problem of obtaining fully-random hashing on a fixed (but unknown to the hash function) set of n keys using O(n) space. As a consequence, we get more efficient implementations of the splitting trick of Dietzfelbinger and Rink [ICALP'09] and the succinct space uniform hashing of Pagh and Pagh [SICOMP'08].
Tornado tabulation hashing is based on a simple method to systematically break dependencies in tabulation-based hashing techniques.
Title: Improved Pattern-Avoidance Bounds for Greedy BSTs via Matrix Decomposition
Greedy BST (or simply Greedy) is an online self-adjusting binary search
tree defined in the geometric view ([Lucas, 1988; Munro, 2000; Demaine,
Harmon, Iacono, Kane, Patrascu, SODA 2009). Along with Splay trees
(Sleator, Tarjan 1985), Greedy is considered the most promising
candidate for being dynamically optimal, i.e., starting with any initial
tree, their access costs on any sequence is conjectured to be within
$O(1)$ factor of the offline optimal. However, despite having received a
lot of attention in the past four decades, the question has remained
elusive even for highly restricted input.
In this talk, we show new bounds on the cost of Greedy in the ``pattern
avoidance'' regime including preorder traversal, postorder traversal,
and deque conjectures. The input sequences in traversal and deque
conjectures are perhaps ``easiest'' in the pattern-avoiding input
classes and yet among the most notorious special cases of the dynamic
optimality conjecture. Our analysis technique yields an optimal bound
for postorder traversal sequence for Greedy.
Key to all these results is to partition (based on the input structures)
the execution log of Greedy into several simpler-to-analyze subsets for
which classical forbidden submatrix bounds can be leveraged. We believe
that this simple method will find further applications in doing
amortized analysis of data structures via extremal combinatorics.
No prior background knowledge is assumed in this talk.
Joint work with Parinya Chalermsook, Manoj Gupta, Wanchote Jiamjitrak,
Nidia Obscura Acosta, and Akash Pareek.
Title: Two-Commodity Flow is Equivalent to Linear Programming
Abstract: Maximum flow is a very well-studied problem, which can be formulated as a linear program. While an almost-linear time algorithm for single-commodity flow is developed, progress for multi-commodity flow is only made by progress in general linear program solvers. It turns out it is not by accident since two-commodity flow problem (2CF) is equivalent to general linear programs (LP). More precisely, we show that any LP (under milder assumptions) can be encoded as a 2CF problem in nearly linear time, with only a polylogarithmic blow-up in problem size and polynomial blow-up in error. Our reduction applies to high-accuracy approximation algorithms and exact algorithms.
Our proof builds upon Itai's polynomial-time reduction from LP to 2CF [Itai JACM'78], improving its reduction time, problem size, and establishing an error bound. In this talk, I will go through the key steps of the reduction algorithm from LP to 2CF. Additionally, I will provide an intuitive error analysis of the solution mapping from a 2CF to LP.
Joint work with Rasmus Kyng and Peng Zhang.
Title: Partial Symmetrization for Eulerian Laplacians
Abstract: Given a weighted, directed graph G, its partial symmetrization replaces each weighted directed edge (u, v) with two weighted edges (u, v) and (v, u) such that the weight of (u, v) is marginally larger than the weight of (v, u). Surprisingly, the obtained graph G’ can be shown to be a useful approximation of G when G is Eulerian.
In this talk, I am going to introduce partial symmetrization, and I will outline the benefit of the added robustness it yields in the context of sparsification. In particular, I will talk about using deterministic tools for sparsifying undirected graphs for obtaining sparsifiers of partially symmetrized Eulerian graphs deterministically. Such sparsifiers are a crucial component of the first deterministic directed Laplacian solver [KMPG22].
No prior knowledge about Laplacian solvers is required.
Title: Almost-Linear Time Algorithms for Incremental Graphs: Cycle Detection, SCCs, s-t Shortest Path, and Maximum & Minimum-Cost Flow
Abstract:
I will give an overview of a new result giving almost-linear time algorithms for many incremental directed graph problems (cycle detection, maintaining SCCs, apx. shortest s-t path, max & min-cost flow). We get our results by developing a data structure that solves the dynamic min-ratio cycle problem deterministically & against any adversary with subpolynomial time per update. Using the L1 IPM of Chen-Liu-Kyng-Peng-Probst-Sachdeva (FOCS 2022), this gives a new and more modular deterministic almost-linear time algorithm for min-cost & maximum flow, and by an extension due fo Brand-Liu-Sidford (STOC 2023), it solves a host of incremental graph problems.
Joint work with Li Chen, Yang Liu, Simon Meierhans, and Max Probst Gutenberg.
Paper draft: http://rasmuskyng.com/papers/2023-nov-15_incrflow_DRAFT.pdf
Title: Almost-Linear Time Algorithms for Incremental Graphs: Cycle Detection, SCCs, s-t Shortest Path, and Maximum & Minimum-Cost Flow
Abstract:
I will give an overview of a new result giving almost-linear time algorithms for many incremental directed graph problems (cycle detection, maintaining SCCs, apx. shortest s-t path, max & min-cost flow). We get our results by developing a data structure that solves the dynamic min-ratio cycle problem deterministically & against any adversary with subpolynomial time per update. Using the L1 IPM of Chen-Liu-Kyng-Peng-Probst-Sachdeva (FOCS 2022), this gives a new and more modular deterministic almost-linear time algorithm for min-cost & maximum flow, and by an extension due fo Brand-Liu-Sidford (STOC 2023), it solves a host of incremental graph problems.
Joint work with Li Chen, Yang Liu, Simon Meierhans, and Max Probst Gutenberg.
Paper draft: http://rasmuskyng.com/papers/2023-nov-15_incrflow_DRAFT.pdf
Title: The Art of Cycle Maintenance
Abstract: I will give an informal blackboard talk on a brand new result giving almost-linear time algorithms for a lot of incremental directed graph problems (cycle detection, maintaining SCCs, apx. shortest s-t path, max & min-cost flow). We get out results by developing a data structure that solves the dynamic min-ratio cycle problem deterministically & against any adversary. By CKL+22, this gives a new deterministic and more modular almost-linear time algorithm for mincost & maximum flow, and by BLS23, it solves a host of incremental graph problems. Joint work with Li Chen, Yang Liu, Simon Meierhans, and Max Probst Gutenberg.
Title: Crash Course on Differential Privacy
Abstract: In this talk, I'll give a crash course on everything you may ever want to know about the basics of differential privacy. My talk will introduce some basic but fundamental concepts in differential privacy and will also touch upon methods and techniques not traditionally covered in an intro lecture on the topic but may be of interest to members of this program such as the sparse vector technique and privacy amplification by subsampling.
Title: The Change-of-Measure Method, Block Lewis Weights, and Approximating Matrix Block Norms
Speaker: Naren Manoj
Abstract: Given a matrix $\mathbf{A} \in \mathbb{R}^{k \times n}$, a partitioning of $[k]$ into groups $S_1,\dots,S_m$, an outer norm $p$, and a collection of inner norms such that either $p \ge 1$ and $p_1,\dots,p_m \ge 2$ or $p_1=\dots=p_m=p \ge 1/\log n$, we prove that there is a sparse weight vector $\mathbf{\beta} \in \mathbb{R}^{m}$ such that $\sum_{i=1}^m \beta_i \cdot \|\mathbf{A}_{S_i}\mathbf{x}\|_{p_i}^p \approx_{1\pm\varepsilon} \sum_{i=1}^m \|\mathbf{A}_{S_i}\mathbf{x}\|_{p_i}^p$, where the number of nonzero entries of $\mathbf{\beta}$ is at most $O_{p,p_i}(\varepsilon^{-2}n^{\max(1,p/2)}(\log n)^2(\log(n/\varepsilon)))$. When $p_1\dots,p_m \ge 2$, this weight vector arises from an importance sampling procedure based on the block Lewis weights, a recently proposed generalization of Lewis weights. Additionally, we prove that there exist efficient algorithms to find the sparse weight vector $\mathbf{\beta}$ in several important regimes of $p$ and $p_1,\dots,p_m$.
Our main technical contribution is a substantial generalization of the change-of-measure method that Bourgain, Lindenstrauss, and Milman used to obtain the analogous result when every group has size $1$. Our generalization allows one to analyze change of measures beyond those implied by D. Lewis's original construction, including the measure implied by the block Lewis weights and natural approximations of this measure.
joint work with Max Ovsiankin. Paper link: https://arxiv.org/abs/2311.10013