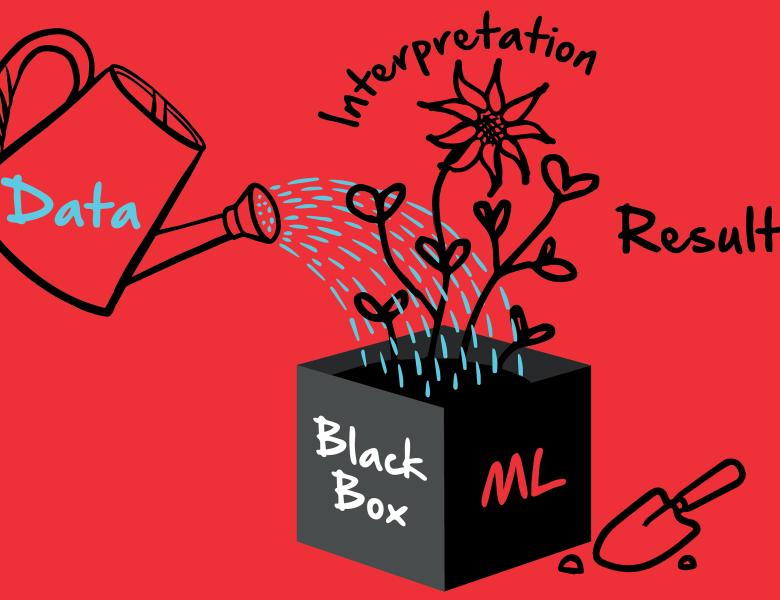
Note: The event time listed is set to Pacific Time.
Recent machine learning models have achieved impressive predictive performance by learning complex functions of many variables, often at the cost of interpretability. This talk will cover recent work aiming to interpret models by attributing importance to features / feature groups for a single prediction. Importantly, the proposed attributions disentangle the importance of features in isolation and the interactions between groups of features. These attributions are shown to yield insights across domains, including an NLP classification task and in understanding cosmological models. Moreover, these attributions can be used during training to directly improve generalization in interesting ways. The talk will place a large emphasis on how to cogently define and evaluate the proposed interpretations.
All scheduled dates:
Upcoming
No Upcoming activities yet