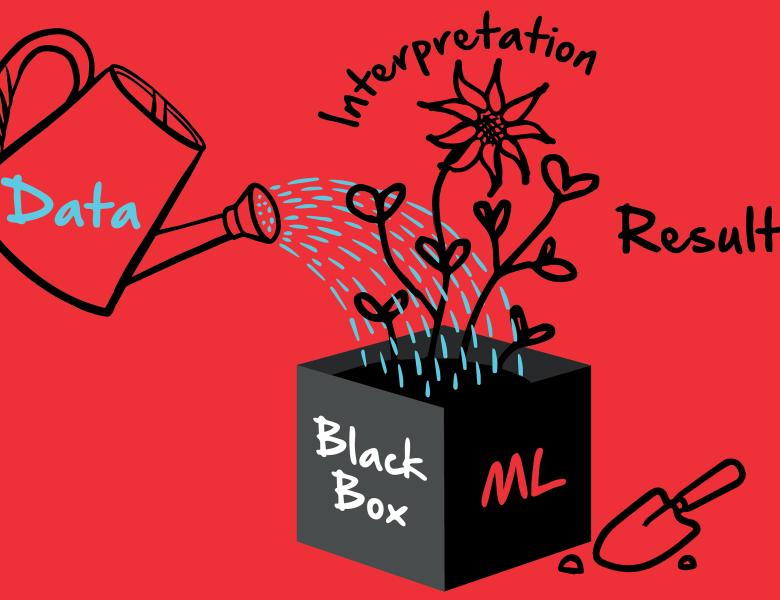
Note: The event time listed is set to Pacific Time.
One strategy for developing interpretable models is to learn representations that are compositional, in that they encode complex concepts as a combination of simple parts. In this talk I will describe two approaches towards this long-standing challenge. The first focuses on causal graph discovery. Standard causal discovery methods must fit a new model whenever they encounter samples from a new underlying causal graph. However, these samples often share relevant information -- for instance, the dynamics describing the effects of causal relations -- which is lost when following this approach. I will present Amortized Causal Discovery, a novel framework that leverages such shared dynamics to learn to infer causal relations from time-series data, and discovers different underlying causal graphs. The second leverages generative models to develop compositional representations of images. We show that training a generative model to imitate drawings across many classes in a "sketch" domain forms representations that are extremely informative for visual tasks. Overall these methods demonstrate how compositional representations can permit robust generalization to novel conditions and domains.
Joint work with Sindy Lowe, David Madras, Max Welling, Mengye Ren, Mike Iuzzolino and Mike Mozer.
All scheduled dates:
Upcoming
No Upcoming activities yet