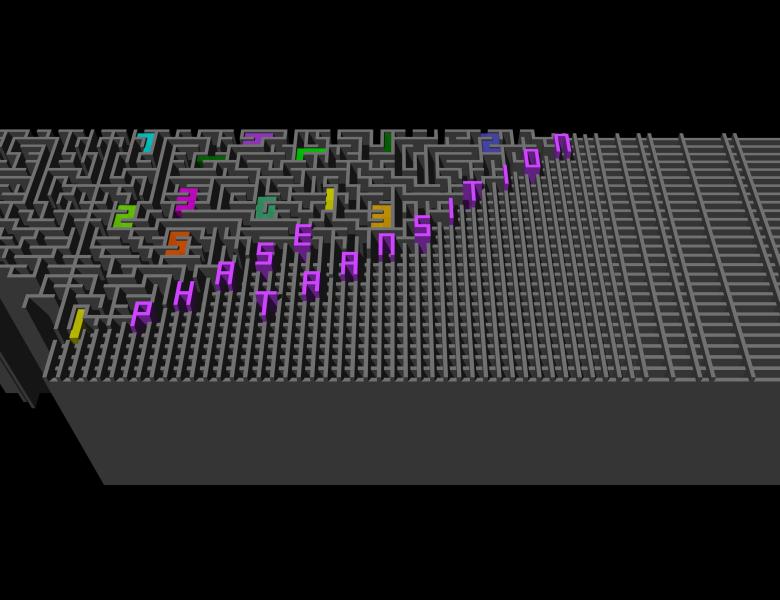
Hamilton Cycles, Polytopes and Markov Chains
In this talk, we introduce a certain polytope, H, induced by a particular discounted Markov decision process corresponding to a given graph G. It has been proved that if the graph G is Hamiltonian, then corresponding to each Hamiltonian cycle in graph G, there exists an extreme point in polytope H, namely, Hamiltonian extreme point. We concentrate on Binomial random graphs G(n,p), where n is the total number of nodes and p is the probability of having an arc between any pair of nodes. We show that the set of all extreme points of polytope H corresponding to a random graph G(n,p) can be partitioned into five subsets and derive the expected cardinality of each of them. These results indicate that the ratio of expected number of Hamiltonian extreme points to all extreme points decays rapidly as it is of the order O(n^n). However, an adaptation of the polytope H, will be shown to have much more favourable ratios of this type. In particular, we constrain H by appropriately designed, efficient linear constraints, to obtain a new polytope WH that is a subset of H. Numerical as well as preliminary theoretical results suggest the following two conjectures on the polytope WH: (i) There exists a fully-polynomial almost uniform sampler to generate extreme points of WH induced by the random graph G(n,p), (ii) The expected value of the proportion of extreme points in WH which are Hamiltonian is polynomially small. At the end of this talk, we explain these two conjectures in more details in provide our approach to tackle them.
All scheduled dates:
Upcoming
No Upcoming activities yet