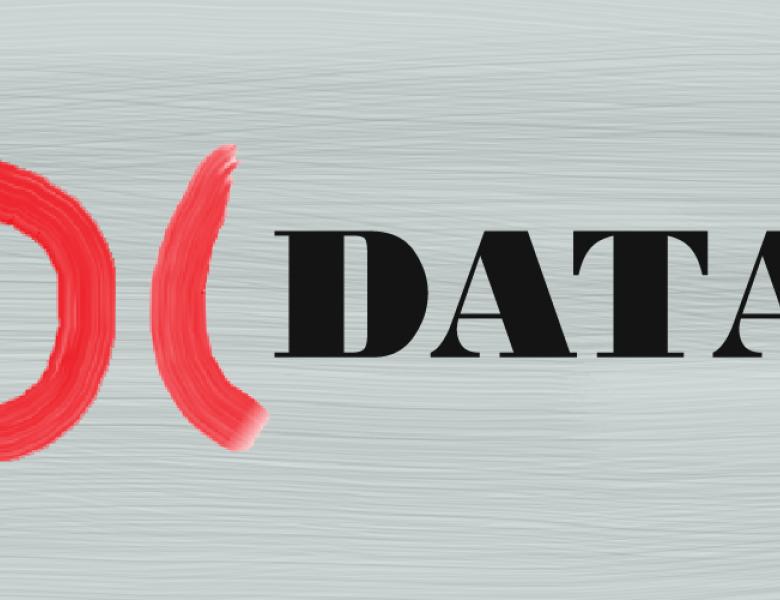
Description
A Block-Coordinate Frank-Wolfe Algorithm, and Applications to Structured Prediction
Coordinate descent on one hand, and the Frank-Wolfe algorithm on the other hand are two of the earliest known first-order methods for convex optimization. Combining these two, we study a new block-coordinate variant of the Frank-Wolfe algorithm for convex optimization with block-separable constraints. Despite its lower iteration cost, we prove similar convergence rate as for the full Frank-Wolfe algorithm. When applied to the structural support vector machine (SVM), this yields an online algorithm that has the same low iteration complexity as stochastic subgradient methods. However, unlike the latter, the block-coordinate Frank-Wolfe algorithm comes with a computable duality gap guarantee, and removes the need to tune any step-size.
Our experiments indicate that this simple algorithm outperforms competing structural SVM solvers.
Joint work with Simon Lacoste-Julien, Mark Schmidt and Patrick Pletscher.
All scheduled dates:
Upcoming
No Upcoming activities yet