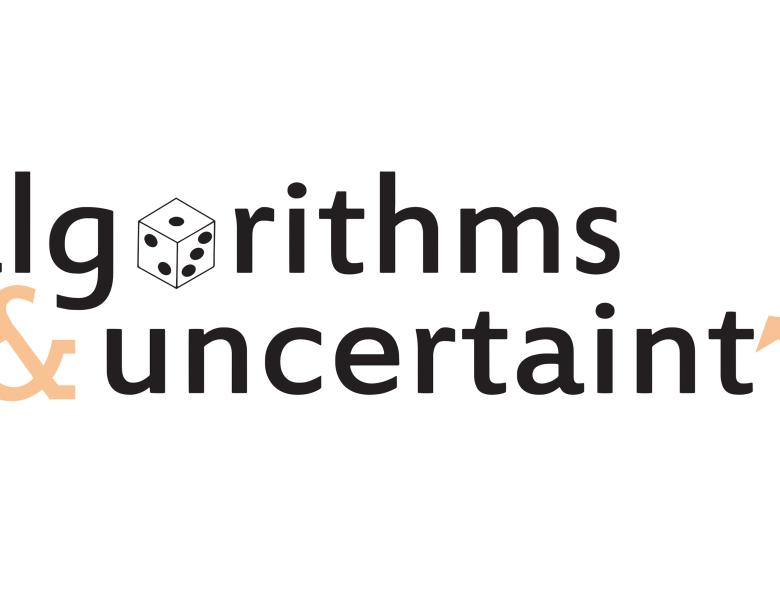
Nir Ailon (Technion Israel Institute of Technology), David Applegate (Google Research, NYC), Sanjeev Arora (Princeton University), Angelos Aveklouris (Eindhoven University of Technology), Nina Balcan (Carnegie Mellon University), Frank Ban (UC Berkeley), Nikhil Bansal (Eindhoven University of Technology), Peter Bartlett (UC Berkeley), Shai Ben-David (University of Waterloo), Eilyan Bitar (Cornell University), Avrim Blum (Carnegie Mellon University), Craig Boutilier (Google and University of Toronto), Kamalika Chaudhuri (UC San Diego), Shuchi Chawla (University of Wisconsin-Madison), Shiri Chechik (Tel Aviv University), Niangjun Chen (California Institute of Technology), Ken Clarkson (IBM Almaden), Edith Cohen (Tel Aviv University and Google Research), Ilan Cohen (Tel Aviv University), Amit Daniely (Hebrew University), Sanjoy Dasgupta (UC San Diego), Soheil Ehsani Banafati (University of Maryland), Uri Feige (Weizmann Institute of Science), Amos Fiat (Tel Aviv University), Shafi Goldwasser (Massachusetts Institute of Technology), Fabrizio Grandoni (IDSIA, University of Lugano), Anupam Gupta (Carnegie Mellon University), Varun Gupta (University of Chicago), Rishi Gupta (Stanford University), Guru Guruganesh (Carnegie Mellon University), MohammadTaghi Hajiaghayi (University of Maryland), Jan Hoffmann (Carnegie Mellon University), Holger Hoos (University of British Columbia), Longbo Huang (Tsinghua University), Sungjin Im (UC Merced), Bart Kamphorst (CWI Amsterdam), Ravi Kannan (Microsoft Research India), Thomas Kesselheim (Max Planck Institute for Informatics and Saarland University), Erica Klarreich, Ilias Koutsoupias (University of Oxford), Robert Krauthgamer (Weizmann Institute), Ravi Kumar (Google), Stefano Leonardi (Sapienza University of Rome), Kevin Leyton-Brown (University of British Columbia), Na Li (Harvard University), Bruce Liang (Princeton University), Pawel Lichocki (Google), Raphael Louca (Cornell University), Konstantin Makarychev (Microsoft Research), Vahab Mirrokni (Google), Ankur Moitra (MIT), Marco Molinaro (PUC-Rio de Janeiro Brazil), Jamie Morgenstern (University of Pennsylvania), Ben Moseley (Washington University in St. Louis), Kamesh Munagala (Duke University), Vaishnavh Nagarajan (Carnegie Mellon University), Debmalya Panigrahi (Duke University), Kameshwar Poolla (UC Berkeley), Kirk Pruhs (University of Pittsburgh), Ben Recht (UC Berkeley), Rhonda Righter (UC Berkeley), Tim Roughgarden (Stanford University), Aviad Rubinstein (UC Berkeley), Piotr Sankowski (University of Warsaw), Saeidreza Seddighin (University of Maryland), Bart Selman (Cornell University), C. Seshadhri (UC Santa Cruz), Sanjit Seshia (UC Berkeley), Or Sheffet (University of Alberta), Sahil Singla (Carnegie Mellon University), Cliff Stein (Columbia University), Xiaorui Sun (Columbia University), Chaitanya Swamy (University of Waterloo), Marc Uetz (University of Twente), Eli Upfal (Brown University), Shai Vardi (California Institute of Technology), Marilena Vendittelli (Sapienza University of Rome), Aravindan Vijayaraghavan (Northwestern University), Maria Vlasiou (Eindhoven University of Technology), Jan Vondrak (Stanford University), David Wajc (Carnegie Mellon University), Jean Walrand (UC Berkeley), Manfred Warmuth (UC Santa Cruz), Matt Weinberg (Princeton University), Colin White (Carnegie Mellon University), Adam Wierman (California Institute of Technology), Qiaomin Xie (University of Illinois at Urbana-Champaign), Bert Zwart (CWI Amsterdam), Jakub Lacki (Sapienza University of Rome)