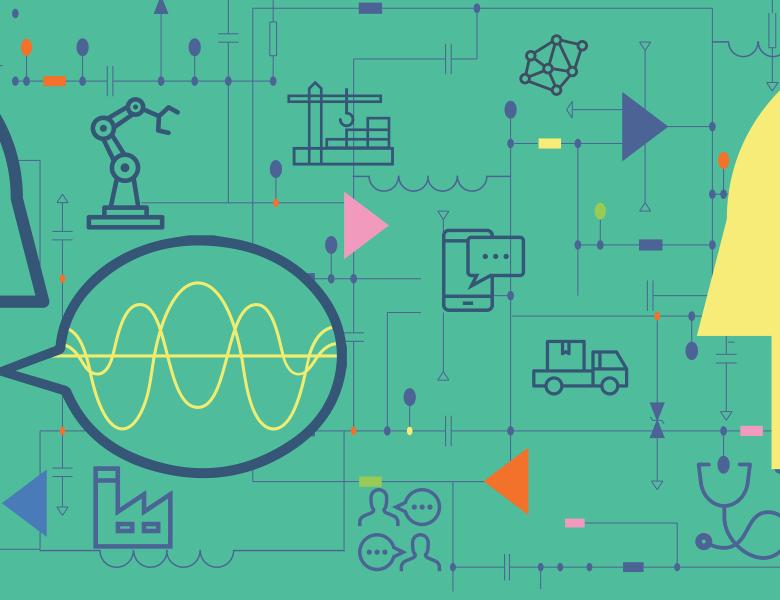
Because of COVID-19, we cannot schedule in-person events on the Berkeley campus through December 2020. This workshop will take place online.
Many of the algorithms and theoretical tools for reinforcement learning assume on-policy data; that is, one can choose a policy and obtain data generated by that policy (either by running the policy or through simulation). In many applications, however, obtaining on-policy data is impossible and all one has is a batch set of data that maybe generated by a nonstationary and even unknown policy. Estimating the value of new policies becomes a hard statistical problem. This workshop attempts to gather some of the tools needed to satisfactorily find good policies with off-policy data, drawing from the statistics and operations research literature, among others. In particular, it will emphasize statistical complexity, confidence bounds and safety guarantees. It will also include recent research on policy certification and robust, reliable policy search. Finally, it will make connections with the system identification and robust control literature from the controls community.
If you require accommodation for communication, information about mobility access, or have dietary restrictions, please contact our Access Coordinator at simonsevents [at] berkeley.edu (subject: Workshop%20accessibility) with as much advance notice as possible.
Rediet Abebe (Harvard), David Abel (Brown University), Alekh Agarwal (Microsoft Research Redmond), Raman Arora (Johns Hopkins University), Luca Baldassarre (Swiss Re), Dimitri Bertsekas (MIT), Jalaj Bhandari (Columbia University), Jeffrey Bohn (Swiss Re), Vivek Shripad Borkar (Indian Institute of Technology Bombay), Sebastien Bubeck (Microsoft Research), Shantanu Prasad Burnwal (IIT Hyderabad), Ana Busic (INRIA), Marco Campi (University of Brescia), Rene Carmona (Princeton University), Lin Chen (UC Berkeley), Brian Christian (UC Berkeley), Munther Dahleh (Massachusetts Institute of Technology), Xiaowu Dai (UC Berkeley), Adithya Munegowda Devraj (University of Florida), Simon Du (University of Washington), Dylan Foster (Massachusetts Institute of Technology (MIT)), Germano Gabbianelli (Universitat Pompeu Fabra), Sumitra Ganesh (JP Morgan), Anupam Gupta (Carnegie Mellon University), Nika Haghtalab (Cornell University), Niao He (University of Illinois at Urbana-Champaign), Rahul Jain (University of Southern California), Nan Jiang (University of Illinois Urbana-Champaign), Chi Jin (Princeton University), Mihailo Jovanovic (University of Southern California), Ravindran Kannan (Microsoft Research India), Amin Karbasi (Yale University), Mikhail Konobeev (University of Alberta), Wouter Koolen (Centrum Wiskunde & Informatica), Akshay Krishnamurthy (Microsoft Research), Jason Lee (Princeton University), Sergey Levine (UC Berkeley), Lihong Li (Google Brain), Yao Liu (Stanford), Tengyu Ma (Stanford University), Shie Mannor (Technion), Aditya Modi (University of Michigan, Ann Arbor), Eric Moulines (Ecole Polytechnique), Vidya Muthukumar (UC Berkeley), Raju Nair (Swiss Re), Joseph Naor (Technion - Israel Institute of Technology), Angelia Nedich (Arizona State University), Gergely Neu (UPF), Ashwin Pananjady (UC Berkeley), Marek Petrik (University of New Hampshire), Ciara Pike-Burke (Universitat Pompeu Fabra), Balaraman Ravindran (IIT Madras), Daniel Russo (Columbia University), Barna Saha (UC Berkeley), Sergey Samsonov (National Research University Higher School of Economics), Bruno Scherrer (INRIA), Dale Schuurmans (University of Alberta), Devavrat Shah (Massachusetts Institute of Technology), Alex Shapiro (Georgia Tech), Roshan Shariff (University of Alberta), Mohamad Kazem Shirani Faradonbeh (University of Florida), Aaron Sidford (Stanford University), Sean Sinclair (Cornell University), R. Srikant (University of Illinois at Urbana-Champaign), Phoebe Sun (Swiss Re), Csaba Szepesvári (University of Alberta, Google DeepMind), Matus Telgarsky (University of Illinois at Urbana-Champaign), Ambuj Tewari (University of Michigan), Claire Tomlin (UC Berkeley), Mathukumalli Vidyasagar (IIT Hyderabad), Stefan Wager (Stanford Graduate School of Business), Mengdi Wang (Princeton University), Ruosong Wang (Carnegie Mellon), Yu-Xiang Wang (UC Santa Barbara), Zhaoran Wang (Northwestern University), Guan Wang (Swiss Re), Chen-Yu Wei (University of Southern California), Qiaomin Xie (Cornell University), Boyi Xie (Swiss Re), Zhuoran Yang (Princeton University), Lin Yang (University of California, Los Angeles), Christina Yu (Cornell University), Huizhen Yu (University of Alberta), Andrea Zanette (Stanford University)