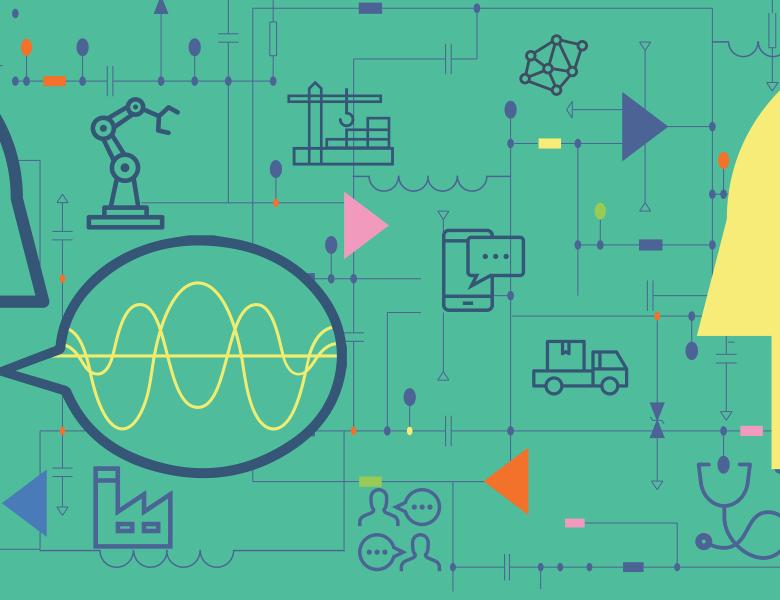
Because of COVID-19, we cannot schedule in-person events on the Berkeley campus through December 2020. This workshop will take place online. It will be open to the public for online participation. Please register to receive the zoom webinar access details.
Reinforcement learning is but one way to model interactions with a dynamic environment. Indeed, online algorithms have a long history in the theoretical computer science community, and many of the concepts (such as regret minimization and competitive ratios) have produced very successful algorithms and design principles (such as exponential weights and choosing actions optimistically). At a high level, this workshop aims to involve the theoretical computer science community by asking which classical online learning tools can be successfully applied to reinforcement learning problems and particularly the problem of exploration. Of particular interest are the tools for designing and analyzing algorithms that are robust to non-stochastic and adversarial data. The bandit problem is well understood in both stochastic and non-stochastic cases, but what is the right approach to "robustify'' exploration in reinforcement learning? One can generalize bandit algorithms to reinforcement learning by considering exploration over a parametric class of Markov decision processes; however, this class is a generalization of linear bandits which is not fully solved, as finite-time, structure-dependent minimax algorithms are unknown. Finally, the "elephant in the room" is to develop flexible methods that scale for a large class of environments and are not sensitive to the so-called realizability assumption.
If you require accommodation for communication, information about mobility access, or have dietary restrictions, please contact our Access Coordinator at simonsevents@berkeley.edu with as much advance notice as possible.
Rediet Abebe (Harvard), Naman Agarwal (Google), Alekh Agarwal (Microsoft Research Redmond), Pierre Alquier (RIKEN), Luca Baldassarre (Swiss Re), Hamsa Bastani (Upenn), Jalaj Bhandari (Columbia University), Jeffrey Bohn (Swiss Re), Vivek Shripad Borkar (Indian Institute of Technology Bombay), Emma Brunskill (Stanford University), Simina Brânzei (Purdue University), Sebastien Bubeck (Microsoft Research), Shantanu Prasad Burnwal (IIT Hyderabad), Ana Busic (INRIA), Marco Campi (University of Brescia), Rene Carmona (Princeton University), Shuchi Chawla (University of Wisconsin-Madison), Lin Chen (Yale University), Brian Christian (UC Berkeley), Dylan Foster (Massachusetts Institute of Technology (MIT)), Germano Gabbianelli (Universitat Pompeu Fabra), David Goldberg (Cornell), Negin Golrezaei (MIT), Steffen Grünewälder (Lancaster University), Anupam Gupta (Carnegie Mellon University), Nika Haghtalab (Cornell University), Niao He (University of Illinois at Urbana-Champaign), Rahul Jain (University of Southern California), Chi Jin (Princeton University), Mihailo Jovanovic (University of Southern California), Sham Kakade (University of Washington), Ravindran Kannan (Microsoft Research India), Emilie Kaufmann (Inria), Mikhail Konobeev (University of Alberta), Wouter Koolen (Centrum Wiskunde & Informatica), Akshay Krishnamurthy (Microsoft Research), Jason Lee (Princeton University), Lihong Li (Google Brain), Michael Littman (Brown University), Yao Liu (Stanford), Tengyu Ma (Stanford University), Yishay Mansour (Tel Aviv Univ and Google Research), Sean Meyn (University of Florida), Aditya Modi (University of Michigan, Ann Arbor), Mehryar Mohri (Google Research & NYU), Eric Moulines (Ecole Polytechnique), Vidya Muthukumar (UC Berkeley), Raju Nair (Swiss Re), Joseph Naor (Technion - Israel Institute of Technology), Angelia Nedich (Arizona State University), Gergely Neu (UPF), Ashwin Pananjady (UC Berkeley), Vianney Perchet (Université Paris Diderot - Paris 7), Marek Petrik (University of New Hampshire), Ciara Pike-Burke (Universitat Pompeu Fabra), Yuval Rabani (The Hebrew University of Jerusalem), Lillian Ratliff (University of Washington), Balaraman Ravindran (IIT Madras), Benjamin Recht (UC Berkeley), Daniel Russo (Columbia University), Barna Saha (UC Berkeley), Sergey Samsonov (National Research University Higher School of Economics), Bruno Scherrer (INRIA), Dale Schuurmans (University of Alberta), Roshan Shariff (University of Alberta), Mohamad Kazem Shirani Faradonbeh (University of Florida), Aaron Sidford (Stanford University), Sean Sinclair (Cornell University), Alex Slivkins (Microsoft Research New York), Phoebe Sun (Swiss Re), Csaba Szepesvári (University of Alberta, Google DeepMind), Éva Tardos (Cornell University), Ambuj Tewari (University of Michigan), Claire Tomlin (UC Berkeley), Mathukumalli Vidyasagar (IIT Hyderabad), Stefan Wager (Stanford Graduate School of Business), Martin Wainwright (UC Berkeley), Zhaoran Wang (Northwestern University), Ruosong Wang (Carnegie Mellon University), Guan Wang (Swiss Re), Mengdi Wang (Princeton University), Chen-Yu Wei (University of Southern California), Boyi Xie (Swiss Re), Lin Yang (University of California, Los Angeles), Zhuoran Yang (Princeton University), Huizhen Yu (University of Alberta), Christina Yu (Cornell University), Yisong Yue (Caltech), Andrea Zanette (Stanford University)