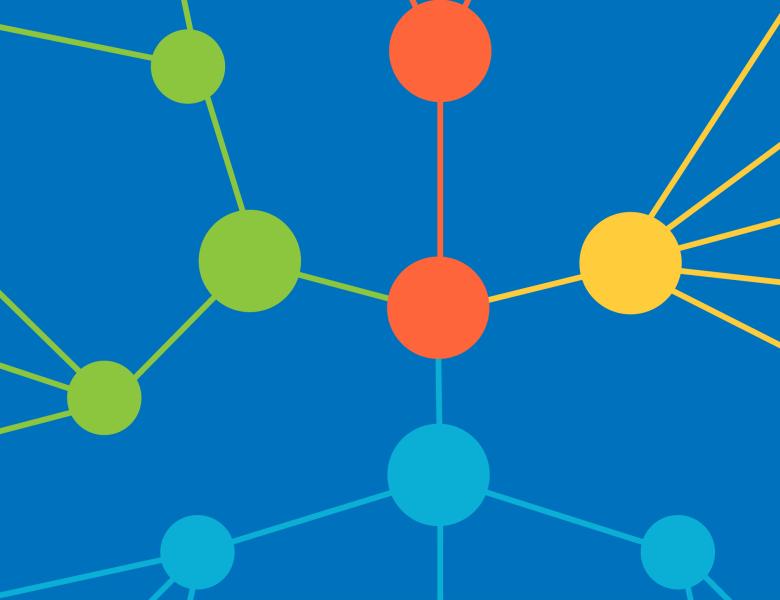
Abstract
Before the introduction of GPT-4, there was a widespread belief that AI was primarily about pattern recognition without genuine understanding. Yet, GPT-4 challenged this perspective, showcasing comprehensive knowledge that went beyond pattern matching. In our talk, we will provide numerous examples that highlight these sparks of AGI in GPT-4. This revelation raises a critical question: is this hint of AGI evident only in expansive models?
To investigate this, we explored the capabilities of a smaller size model geared towards code generation. We demonstrate that with high-quality data, the demand for expansive datasets and a multitude of parameters lessens. The outcome was a 1.3B size model, which not only met or exceeded the performance of existing open-source models but did so utilizing a mere 1/1000th of compute in training. Moreover, we will discuss specific emergent properties observed in the model after its fine-tuning on coding exercises.