Image
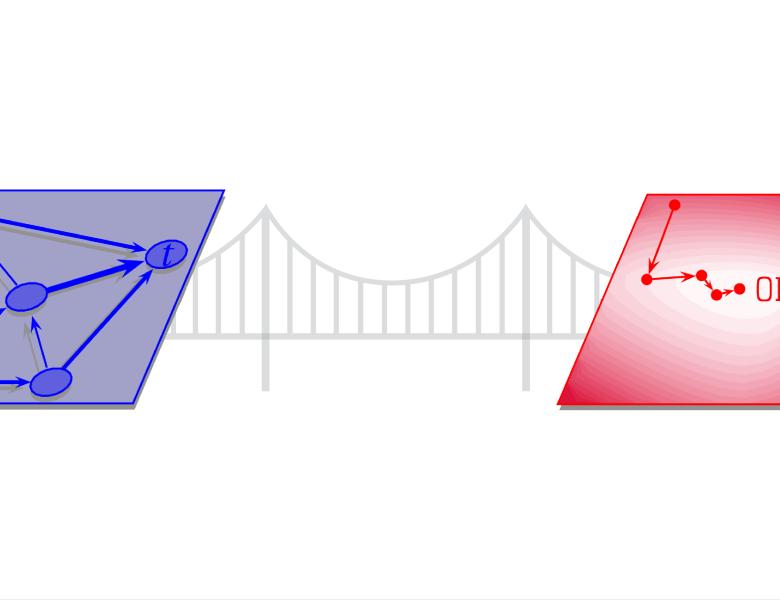
In the last few years, Michael Cohen has developed faster algorithms for many important problems in optimization such as Laplacian, directed Laplacian, min cost flow, geometric median, matrix scaling, linear/lp regression, clustering,
In the first few minutes of the talk, I will review some of the great achievements in optimization by Michael.
Then, I will present his yet another great achievement, the first input sparsity polynomial time algorithm for lp regression for 1<p<inf. Except for l2 regression, this is the first problem that we can solve in input sparsity time with running time polynomial to log(1/eps).
Joint work with Sébastien Bubeck, Michael Cohen and Yuanzhi Li.