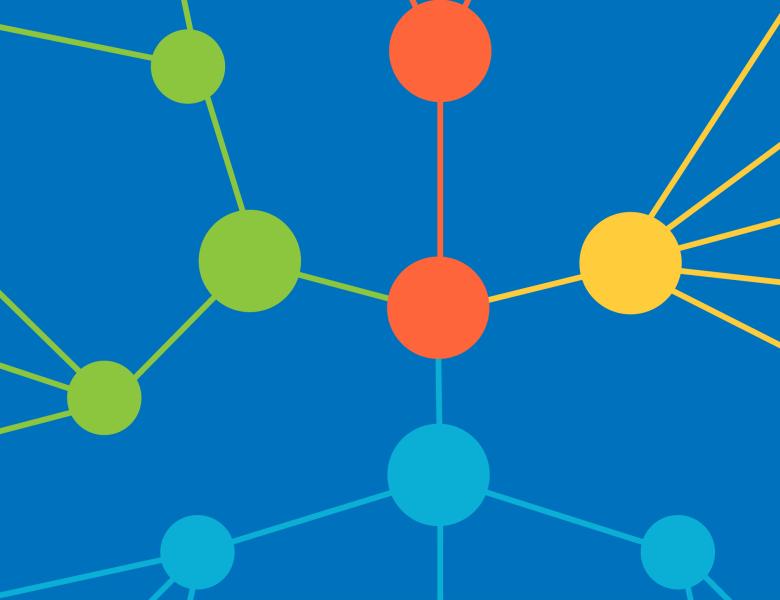
Abstract
Recent advances in large ML models – in language and other domains – have empirically highlighted how performance can follow simple trends as a function of basic knobs including amount of training data, model size, and amount of compute. Can we understand the origins of these phenomenological forms and relate them back to more fundamental quantities? I will describe some of our findings that draw from deep learning theory and strive to be consistent with experiments. We propose a classification for different scaling regimes based on what drives the improvements in performance, and we identify regimes where scaling exponents empirically and theoretically can take on universal values. I’ll also touch on some findings from large-scale evaluation efforts such as BIG-bench and discuss directions for future research.